HoloPart Enables Complete 3D Part Segmentation
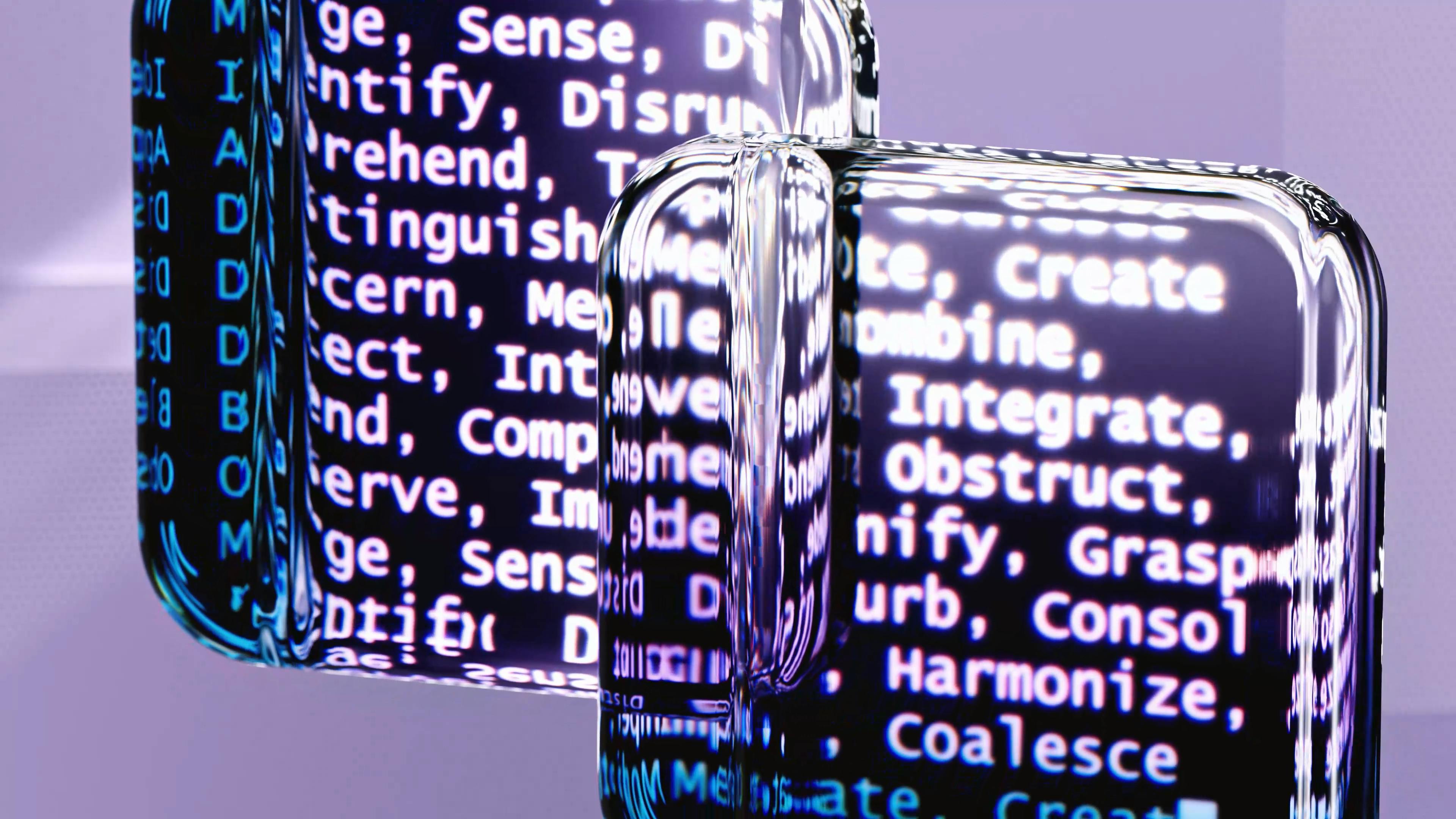
Top post
Next-Generation 3D Modeling: HoloPart Enables Complete Part Segmentation
The world of 3D modeling and analysis is advancing rapidly. A new method called "3D part amodal segmentation" is in focus, which has the potential to fundamentally change the way we interact with 3D objects. This technique allows a 3D model to be broken down into its semantically meaningful individual parts, even if these parts are partially obscured or overlapped by other objects. This opens up new possibilities for applications in areas such as 3D content creation, animation, material assignment, and even robotics.
Previous methods for 3D part segmentation have focused primarily on identifying visible surface areas. This limits their applicability, as hidden parts cannot be recognized and therefore cannot be used for further processing steps. 3D part amodal segmentation overcomes this limitation by reconstructing the complete geometry of individual parts, regardless of their visibility.
A promising approach in this area is HoloPart, a two-stage process that addresses the challenges of reconstructing occluded 3D geometry, maintaining global shape consistency, and handling diverse shapes with limited training data. In the first step, HoloPart utilizes existing 3D part segmentation methods to obtain initial, incomplete part segments. In the second step, a diffusion-based model is used to complete these segments into full 3D parts.
The architecture of HoloPart is specifically designed to capture fine-grained part geometries and ensure overall shape consistency. Local attention enables the precise reconstruction of individual parts, while global shape context attention mechanisms ensure the coherence of the entire 3D model. This approach allows even complex shapes to be segmented with high accuracy.
To evaluate the performance of HoloPart, new benchmarks were developed based on the ABO and PartObjaverse-Tiny datasets. The results show that HoloPart significantly outperforms existing shape completion methods. By combining HoloPart with established segmentation techniques, promising results can be achieved in 3D part amodal segmentation.
The development of HoloPart opens up new possibilities for 3D modeling and analysis. Applications such as geometry editing, animation, and material assignment can benefit from the ability to accurately identify and manipulate even hidden parts. Research in this area is dynamic and promising, and it is expected that 3D part amodal segmentation will play a key role in 3D computer graphics and vision in the future.
For companies like Mindverse, which specialize in AI-powered content creation and analysis, HoloPart offers enormous potential. Integrating this technology into existing platforms could significantly expand the possibilities of automated 3D modeling and editing, opening new avenues for the development of innovative applications.
Bibliography: - Yang, Y., Guo, Y.-C., Huang, Y., Zou, Z.-X., Yu, Z., Li, Y., Cao, Y.-P., & Liu, X. (2025). HoloPart: Generative 3D Part Amodal Segmentation. arXiv preprint arXiv:2504.07943. - https://chatpaper.com/chatpaper/zh-CN?id=4&date=1744300800&page=1 - https://huggingface.co/papers/2411.07184 - https://arxiv.org/abs/2107.07464 - https://paperswithcode.com/task/amodal-instance-segmentation - https://www.researchgate.net/publication/384213590_Generative_3D_Part_Assembly_via_Part-Whole-Hierarchy_Message_Passing - https://openaccess.thecvf.com/content/CVPR2024/papers/Ozguroglu_pix2gestalt_Amodal_Segmentation_by_Synthesizing_Wholes_CVPR_2024_paper.pdf - https://zhixuanli.github.io/ - https://arxiv.org/abs/2402.17464 - https://www.researchgate.net/figure/Amodal-instance-segmentation-results-on-natural-images-Our-CSDNet-learns-to-predict-the_fig4_354900393