Advances in 3D Scene Captioning with Contrastive Learning
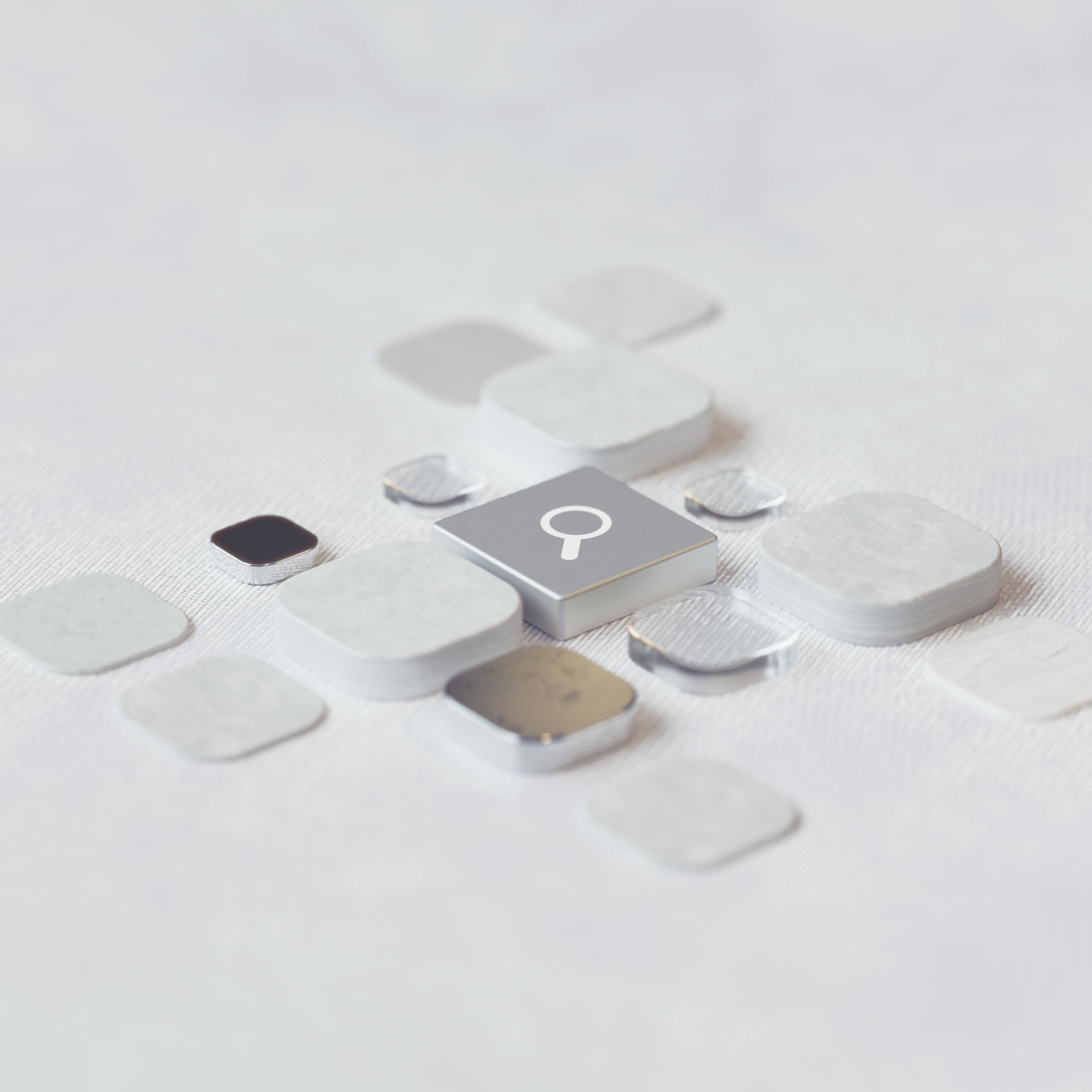
Top post
Understanding and Describing 3D Scenes: Advances in 3D Captioning with Contrastive Learning
The automatic description of 3D scenes, also known as 3D captioning, is a complex research field in Artificial Intelligence. The goal is to develop algorithms that can capture and describe the content of three-dimensional scenes in natural language. This technology has far-reaching applications, from assisting visually impaired people to automated content analysis in virtual environments. However, its implementation presents challenges for research, particularly due to the specific characteristics of 3D data.
One of the biggest hurdles lies in the representation of 3D scenes. Point clouds, a common representation form, are often characterized by their sparse data. Unlike images, which have a dense pixel structure, point clouds consist of a collection of individual points in 3D space. This sparse data structure makes it difficult to extract relevant information for describing the scene.
Another critical point is the effective linking of visual and linguistic information. Previous approaches in 3D captioning have struggled to establish a robust connection between the 3D representation of the scene and the generated linguistic description. This has led to inaccurate or incomplete descriptions that do not adequately reflect the actual scene content.
New research results, however, show promising progress in this area. An innovative approach called 3D CoCa (Contrastive Captioners) combines contrastive learning with image captioning generation in a single architecture. This approach leverages the power of pre-trained vision-language models like CLIP to extract semantic information from the 3D scenes. A spatially-aware 3D scene encoder captures the geometric context, while a multimodal decoder generates the actual description.
In contrast to previous two-stage methods that rely on explicit object proposals, 3D CoCa optimizes the contrastive and descriptive objectives jointly in a unified feature space. This eliminates the need for external detectors or manually created proposals. This joint training improves spatial understanding and semantic grounding by aligning 3D and text representations.
Evaluations on established benchmarks such as ScanRefer and Nr3D show that 3D CoCa significantly outperforms the current state-of-the-art. The results demonstrate that the combination of contrastive learning and 3D captioning in a unified framework leads to a significant improvement in description quality.
The further development of 3D captioning methods like 3D CoCa opens up new possibilities for interacting with and understanding 3D scenes. The ability to automatically and accurately describe 3D content is an important step towards a seamless integration of virtual and real worlds.
Bibliography: - https://paperreading.club/page?id=299359 - https://arxiv.org/abs/2205.01917 - https://www.researchgate.net/publication/360384069_CoCa_Contrastive_Captioners_are_Image-Text_Foundation_Models - https://neurips.cc/virtual/2023/poster/72554 - https://arxiv.org/html/2306.07915v5 - https://dl.acm.org/doi/10.5555/3618408.3619579 - https://proceedings.mlr.press/v202/qi23a/qi23a.pdf - https://proceedings.neurips.cc/paper_files/paper/2023/file/92369a01fbe8046a093746389b2c413e-Paper-Conference.pdf - https://www.sciencedirect.com/science/article/abs/pii/S0923596524000304 - https://dl.acm.org/doi/10.5555/3666122.3668151