ZipIR: A Novel Framework for Efficient High-Resolution Image Restoration
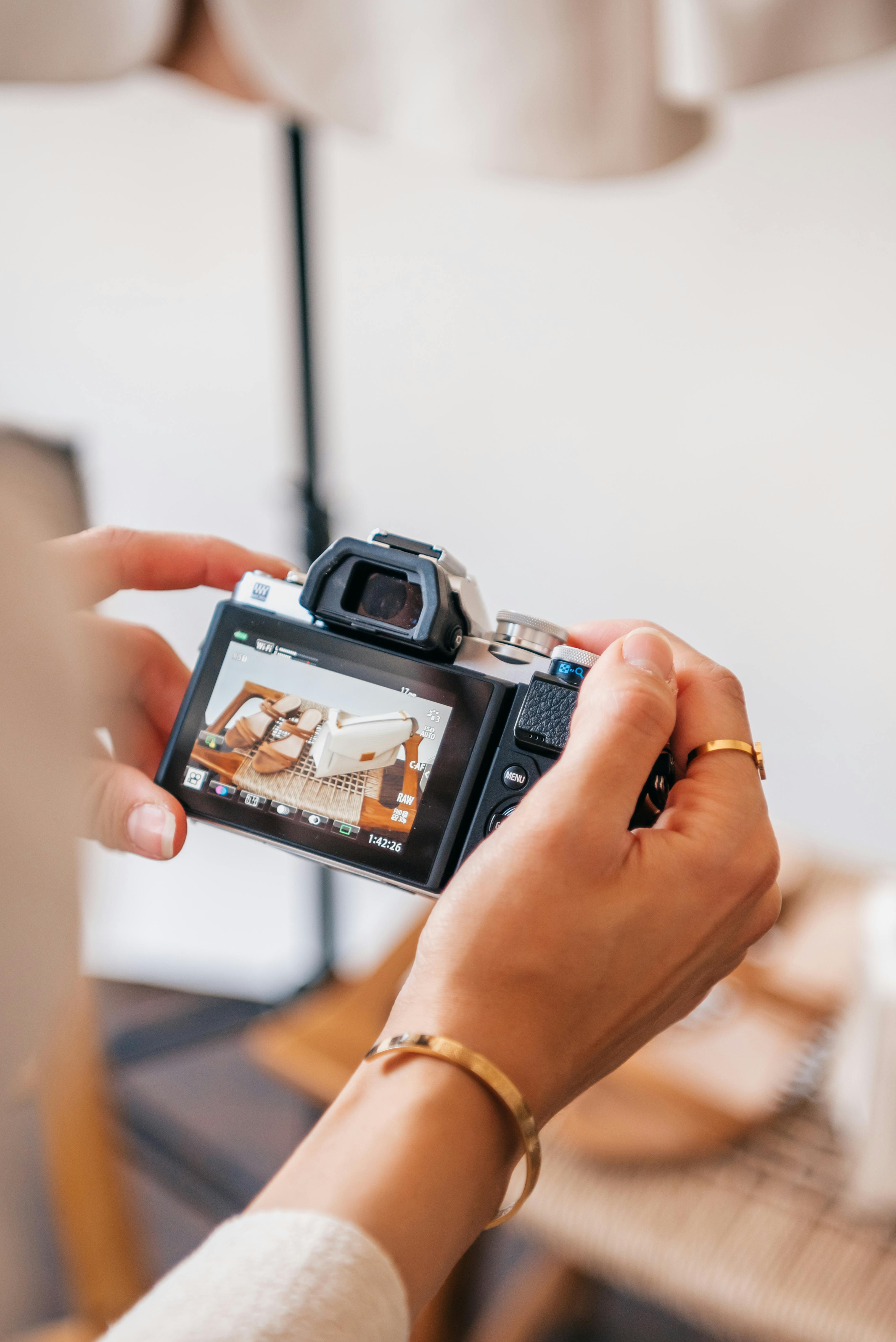
Top post
Image Restoration in Ultra-HD: ZipIR Sets New Standards
The restoration of images, particularly in high resolutions, presents a complex challenge. Contamination, damage, or artifacts impair image quality and hinder interpretation. Artificial intelligence, specifically generative models, offers promising approaches to address this task. Recently, diffusion models, in particular, have made considerable progress due to their ability to restore semantic details and local structures. However, applying these models to ultra-high-resolution images pushes their limits due to the high computational cost of long-range attention mechanisms. A compromise between quality and efficiency is often unavoidable.
A new development promises to overcome this hurdle: ZipIR, an innovative framework that optimizes efficiency, scalability, and long-range modeling for image restoration in high resolutions. The core of ZipIR lies in the use of a highly compressed latent representation, which compresses the image by a factor of 32. This significantly reduces the number of spatial tokens and enables the use of powerful models like the Diffusion Transformer (DiT).
To facilitate diffusion in the latent space, ZipIR uses a specially designed Latent Pyramid VAE (LP-VAE). This architecture structures the latent space into sub-bands, making the processing and manipulation of image information more efficient. By training with full images with resolutions up to 2K, ZipIR surpasses existing diffusion-based methods in terms of speed and quality when restoring high-resolution images from heavily degraded inputs.
The Advantages of the Latent Pyramid Structure
The LP-VAE architecture plays a crucial role in ZipIR's performance. By dividing the latent space into a pyramid structure, the complexity of the image information is reduced, and diffusion in the latent space is optimized. This allows for more efficient processing and enables the application of powerful transformer models like DiT, which would otherwise be unsuitable for high-resolution images due to their high computational cost.
Overcoming the Limits of Conventional Methods
Conventional image restoration methods often reach their limits with ultra-high-resolution images. The high computational cost and the complexity of the image information require efficient data processing strategies. ZipIR addresses these challenges by combining a compressed latent representation with the LP-VAE architecture and the powerful DiT model. This approach enables a significant improvement in efficiency and scalability without compromising the quality of the restoration.
Outlook and Potential
ZipIR represents a promising advance in the field of image restoration. The ability to restore high-resolution images efficiently and with high quality opens up new possibilities in various application areas, from medical imaging to digital art. Future research could focus on further optimizing the LP-VAE architecture and adapting the framework to specific restoration tasks. The development of specialized hardware solutions could further enhance ZipIR's performance and enable its application to even higher resolutions.
Bibliography: Yu, Y., Zheng, H., Zhang, Z., Zhang, J., Zhou, Y., Barnes, C., Liu, Y., Xiong, W., Lin, Z., & Luo, J. (2025). ZipIR: Latent Pyramid Diffusion Transformer for High-Resolution Image Restoration. arXiv preprint arXiv:2504.08591. Zamir, S. W., Arora, R., Khan, S., Hayat, M., Khan, F. S., Yang, M. H., & Shao, L. (2022). Restormer: Efficient transformer for high-resolution image restoration. In Proceedings of the IEEE/CVF Conference on Computer Vision and Pattern Recognition (pp. 12997-13006). Huang, Z., Wang, Y., Zhang, Y., & Wang, Y. (2024). Latent Diffusion Enhanced Rectangle Transformer for Hyperspectral Image Restoration. arXiv preprint arXiv:2403.18446.