BIOMEDICA: A Large-Scale Open Biomedical Image-Text Dataset and Pretrained Models
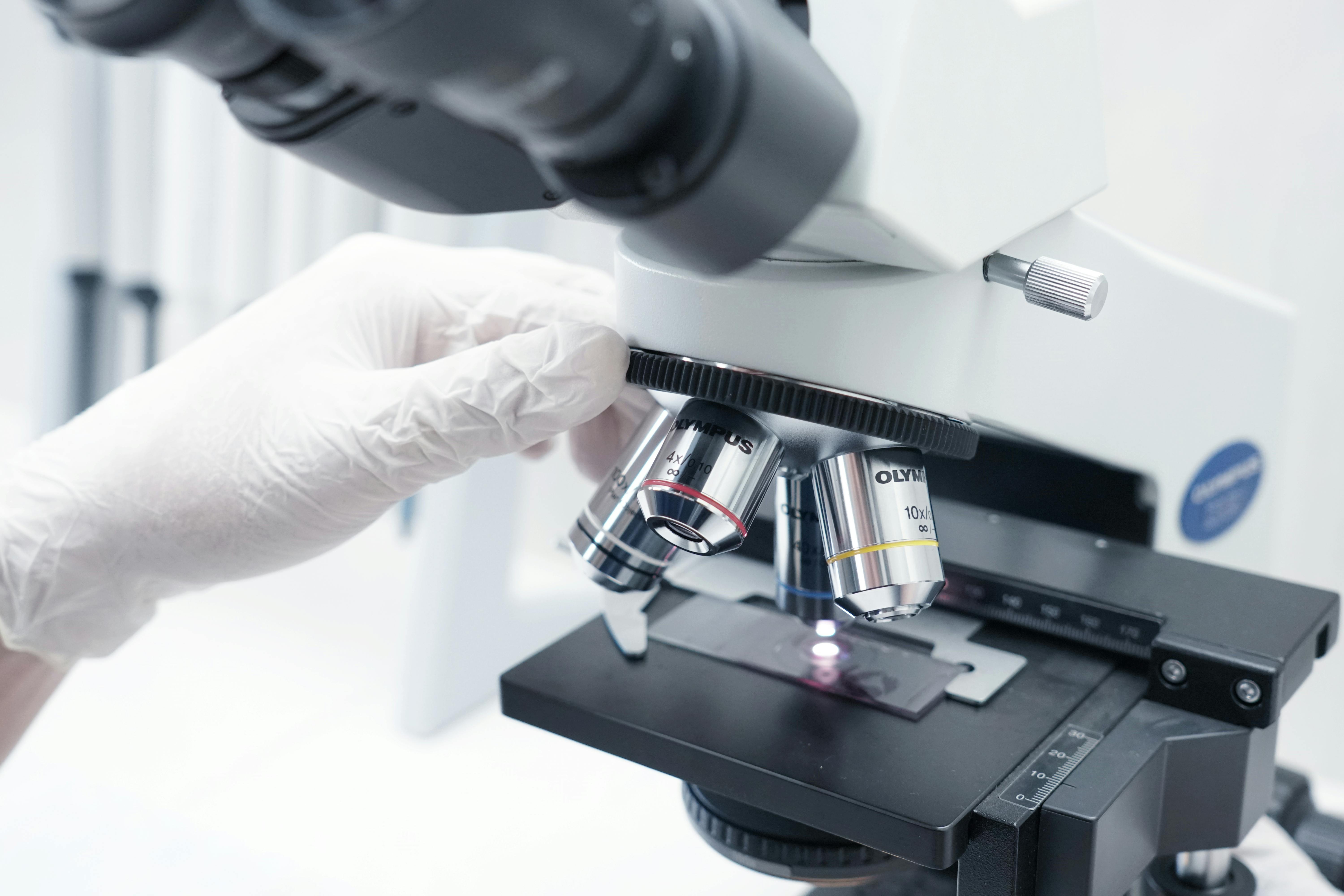
Top post
Unlocking Biomedical Knowledge: BIOMEDICA – An Open Image-Text Archive
The rapid development of Vision-Language Models (VLMs) is revolutionizing the processing and interpretation of image and text data. Driven by large and diverse multimodal datasets, these models demonstrate impressive capabilities in understanding and generating content. However, progress in the biomedical field lags behind due to a lack of freely accessible, annotated datasets that cover the full breadth of biological and medical knowledge.
Existing datasets often focus on specific sub-domains, leaving untapped the wealth of information hidden within scientific publications. To address this gap, BIOMEDICA has been developed – a scalable open-source framework for extracting, annotating, and serializing the entire publicly accessible subset of PubMed Central.
A Vast Archive of Biomedical Knowledge
BIOMEDICA offers a comprehensive archive of over 24 million unique image-text pairs from over 6 million articles. In addition to image and text data, metadata and expert-guided annotations are also provided. This detailed information allows for a deeper understanding of the relationships between images and texts and facilitates the development of powerful VLMs.
However, the sheer size of the dataset – 27 TB – poses a challenge for processing and utilization. To simplify working with BIOMEDICA, the BMCA-CLIP models have been developed. These CLIP-like models are continuously pre-trained via streaming on the BIOMEDICA dataset, eliminating the need for local download of the entire dataset.
BMCA-CLIP: Outstanding Performance with Reduced Computational Cost
The BMCA-CLIP models achieve state-of-the-art results in various biomedical domains. In tests across 40 tasks, ranging from pathology and radiology to ophthalmology and dermatology, as well as surgery, molecular biology, parasitology, and cell biology, the models surpassed previous performance. Particularly impressive is the performance in zero-shot classification, with an average improvement of 6.56% and peaks of 29.8% in dermatology and 17.5% in ophthalmology.
Furthermore, the BMCA-CLIP models demonstrate improved image-text retrieval performance – all with ten times lower computational cost compared to previous models. This gain in efficiency makes the use of VLMs in the biomedical field significantly more practical and opens up new possibilities for research and application.
Open Source for the Research Community
To promote the reproducibility of research results and collaboration within the community, both the code and the dataset of BIOMEDICA have been made publicly available. This openness allows researchers worldwide to access the resources, develop their own models, and expand the boundaries of biomedical knowledge.
BIOMEDICA represents a significant step towards powerful and accessible VLMs for biomedicine. The project has the potential to accelerate research and development in this field and ultimately lead to improved diagnoses, therapies, and a deeper understanding of biological and medical processes.
Bibliographie: https://arxiv.org/html/2406.09454v1 https://arxiv.org/abs/2406.09454 https://pmc.ncbi.nlm.nih.gov/articles/PMC11384335/ https://papers.miccai.org/miccai-2024/328-Paper2320.html https://medicalxpress.com/news/2024-11-source-ai-biomedical-images-text.html https://openreview.net/pdf?id=KRLUvxh8uaX