RepVideo: Enhanced Representations Improve AI Video Generation
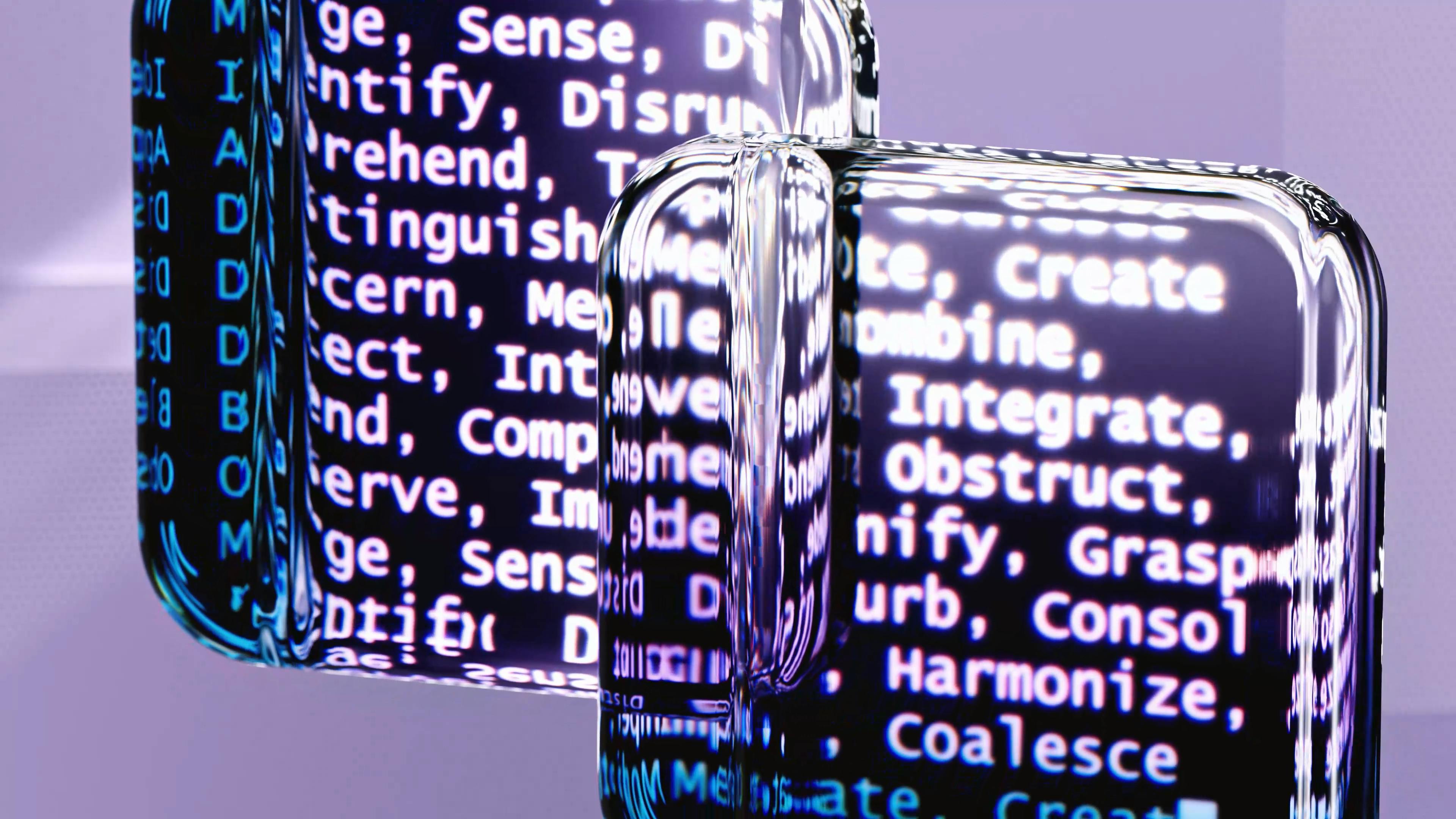
Top post
Representations in Focus: The Challenges of Video Generation
Video generation has made remarkable progress with the introduction of diffusion models, significantly improving the quality of generated videos. However, recent research has primarily focused on scaling model training, while the direct impact of representations on the video generation process has received less attention. A new approach, RepVideo, promises a remedy.
Representations in Focus: Challenges of Video Generation
Current research shows that the features in the intermediate layers of diffusion models exhibit significant variations in the attention maps. These variations lead to unstable semantic representations and contribute to cumulative differences between the features. The consequence: The similarity between neighboring frames is reduced, which negatively affects temporal coherence. In other words, the transitions between the individual frames of a video appear unnatural and jerky.
RepVideo: A New Approach for Enhanced Video Quality
To address these challenges, RepVideo has been developed, an enhanced representation framework for text-to-video diffusion models. The core of the approach lies in the accumulation of features from neighboring layers to form enriched representations. This approach enables the capture of more stable semantic information. The resulting representations then serve as input for the attention mechanism. This improves the semantic expressiveness while ensuring the consistency of features across neighboring frames.
Enhanced Spatial Representation and Temporal Coherence
Comprehensive experiments demonstrate that RepVideo not only significantly improves the ability to generate accurate spatial appearances, such as capturing complex spatial relationships between multiple objects, but also optimizes temporal coherence in video generation. This means that the generated videos not only depict more realistic objects and scenes but also exhibit smoother and more natural motion sequences.
RepVideo in the Context of Current Developments
RepVideo joins a series of innovations in the field of AI-powered video generation. While many approaches focus on scaling models and computing power, RepVideo relies on a refined analysis and utilization of internal representations. This approach opens up new possibilities for improving the quality and coherence of generated videos and could make a significant contribution to the further development of the field. Especially for companies like Mindverse, which develop customized AI solutions, RepVideo offers the potential to significantly increase the performance of applications such as chatbots, voicebots, and AI search engines. Through improved video generation, these applications can create even more realistic and engaging content, thus optimizing the user experience.
Mindverse: AI Partner for Innovative Solutions
For Mindverse, a German company specializing in AI-powered content creation, innovations like RepVideo are of particular interest. As a provider of an all-in-one platform for AI text, images, and research, as well as a developer of customized chatbots, voicebots, and AI search engines, Mindverse benefits from advances in video generation. These technologies enable Mindverse to offer its customers even more powerful and innovative solutions.
Bibliography
- https://huggingface.co/papers/2501.08994
- https://huggingface.co/akhaliq/activity/all
- https://www.sciencedirect.com/science/article/abs/pii/S0031320324004953
- https://arxiv.org/abs/2408.06248
- https://www.ecva.net/papers/eccv_2024/papers_ECCV/papers/05996.pdf
- https://www.chatpaper.com/chatpaper/fr?id=4&date=1736956800&page=1
- https://openaccess.thecvf.com/content/CVPR2022/papers/Guo_Cross-Architecture_Self-Supervised_Video_Representation_Learning_CVPR_2022_paper.pdf
- https://www.semanticscholar.org/paper/8e7aeb2d27e111ec0dfaedb5a607ae1249d161a9
- https://dl.acm.org/doi/10.1145/2030613.2030646
- https://arxiv.org/html/2412.03603v1