Video SimpleQA: A New Benchmark for Evaluating Factuality in Large Video Language Models
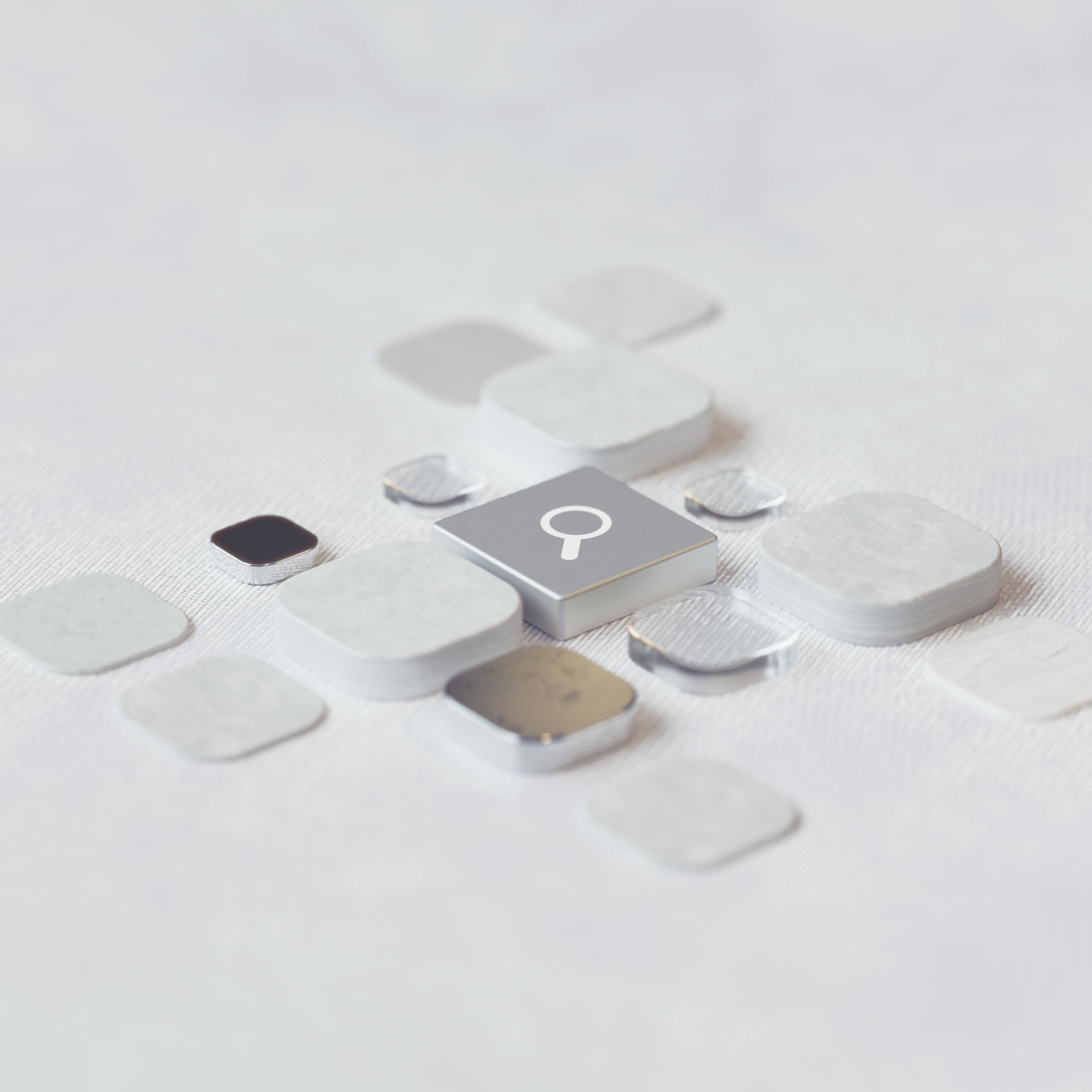
Top post
Fact Verification for AI Video Systems: Video SimpleQA Sets New Standards
The rapid development of large video language models (LVLMs) opens up fascinating possibilities in the field of multimodal understanding. However, verifying the factual accuracy of these models, especially in the context of videos, presents a significant challenge. A new benchmark called Video SimpleQA aims to close this gap and raise the evaluation of LVLMs' factuality to a new level.
Video SimpleQA distinguishes itself from existing video benchmarks through five core features:
- Necessity of External Knowledge: Answering the questions requires the integration of knowledge that goes beyond the explicit content of the video. - Fact-Based Questions: The questions focus on objective, undisputed events or relationships and avoid subjective interpretations. - Clear and Concise Answers: The answers are unambiguous and clearly formulated as correct, enabling automated evaluation by LLM-based evaluation frameworks with minimal variance. - Verification by External Sources: All annotations are rigorously validated against reliable external sources to ensure reliability. - Requirement of Temporal Reasoning: The annotated question types encompass both the understanding of static single frames and dynamic temporal reasoning, thereby explicitly evaluating the factuality of LVLMs considering long-term dependencies.As part of the development of Video SimpleQA, 41 state-of-the-art LVLMs were comprehensively evaluated. The results of this evaluation reveal some interesting insights:
A central finding is that current LVLMs, especially open-source models, exhibit significant deficits in factual accuracy. Even the most powerful model, Gemini-1.5-Pro, only achieves an F-score of 54.4%. This highlights the urgent need for improvements in this area.
Furthermore, test-time computation methods show no significant performance gains. This suggests that improving factuality through post-hoc calculations is subject to fundamental limitations.
Retrieval-Augmented Generation, meaning the extension of LVLMs with retrieval mechanisms, does lead to consistent improvements, but at the cost of increased inference time. This presents developers with the challenge of finding an optimal compromise between efficiency and performance.
Video SimpleQA thus provides a valuable foundation for the further development of LVLMs. By focusing on factual accuracy and providing a comprehensive benchmark, Video SimpleQA contributes to increasing the reliability and trustworthiness of AI-powered video systems. The insights from the evaluations offer important clues for future research and the development of more robust and fact-based LVLMs.
For Mindverse, as a provider of AI-based content solutions, these developments are of particular importance. Improving the factual accuracy of AI models is a central aspect for the development of trustworthy and reliable AI applications. Mindverse closely follows these developments and integrates the latest research findings into its own products and services to always offer customers the best possible solutions. The development of customized AI solutions, such as chatbots, voicebots, AI search engines, and knowledge systems, directly benefits from advances in the fact-checking and accuracy of LVLMs. This enables the creation of AI systems that are not only intelligent but also factually correct and trustworthy.
Bibliography
Cao et al. (2025). Video SimpleQA: Towards Factuality Evaluation in Large Video Language Models. arXiv preprint arXiv:2503.18923.
Lin et al. (2021). TruthfulQA: Measuring How Models Mimic Human Falsehoods. arXiv preprint arXiv:2109.07958.