URSA: A New Approach to Verification in Multimodal Mathematical Reasoning
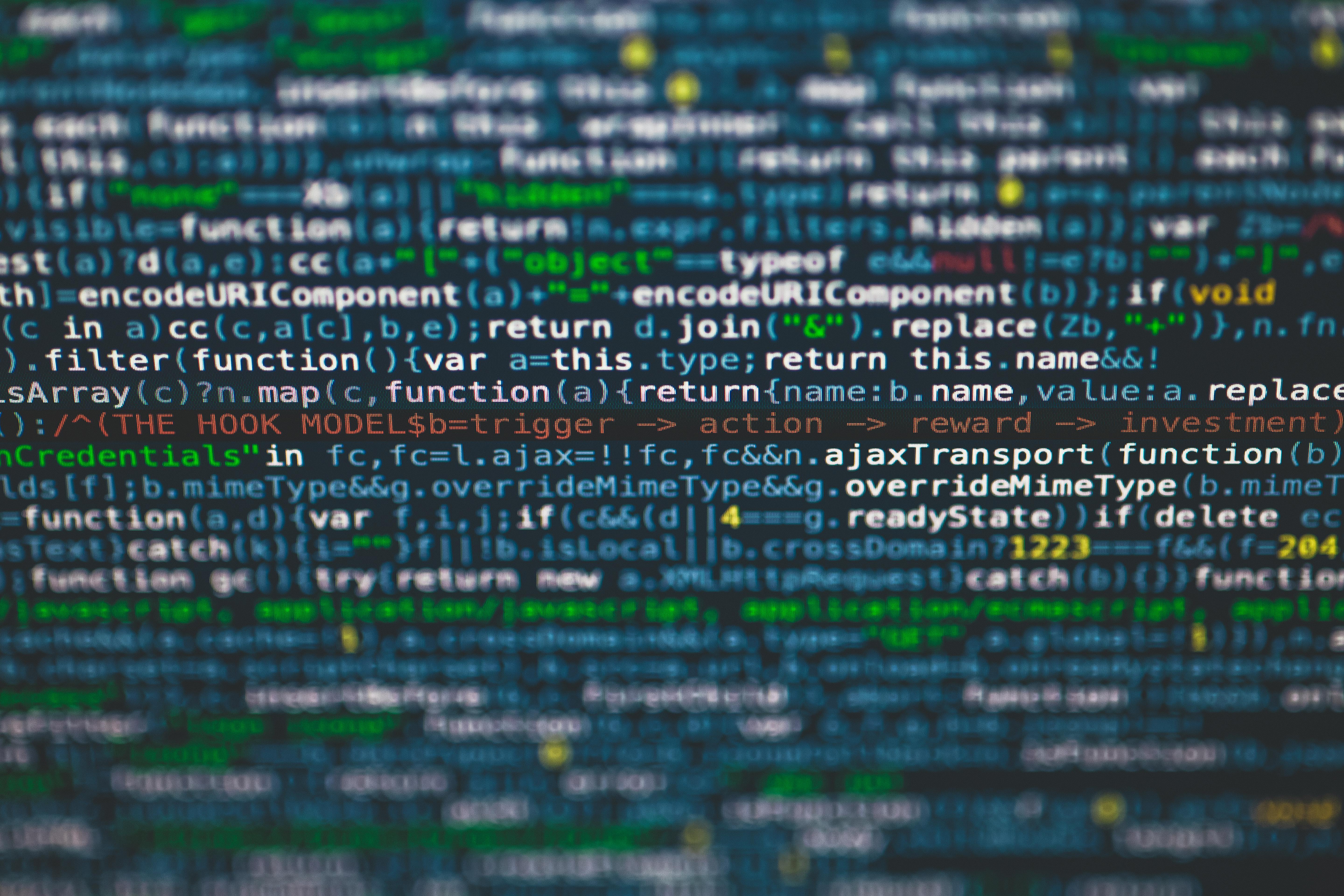
Top post
Chain-of-Thought Reasoning in Multimodal Mathematics: URSA – A New Approach to Understanding and Verification
The ability to solve mathematical problems is one of the core competencies of Artificial Intelligence (AI). Particularly in the field of multimodal mathematical reasoning, which incorporates both text and images, significant progress has been made recently. A promising approach is the so-called Chain-of-Thought (CoT) reasoning, where the AI reveals its solution process step by step, similar to a human. A new model called URSA now demonstrates how CoT reasoning in multimodal mathematics can not only be understood but also verified.
The Challenge of Data Scarcity and URSA's Solution
A major obstacle in the development of AI models for mathematical reasoning is the scarcity of high-quality training data, especially for CoT. URSA addresses this challenge with a three-part strategy: CoT distillation, trajectory format rewriting, and format unification. This method enables the creation of MMathCoT-1M, a comprehensive dataset for fine-tuning multimodal mathematical CoT models.
URSA-7B: A State-of-the-Art Model
The URSA-7B model, trained on MMathCoT-1M, achieves state-of-the-art results for models of its size in various multimodal mathematical benchmarks. This success underscores the effectiveness of the data synthesis approach and training procedure chosen by the URSA developers.
DualMath-1.1M and URSA-RM-7B: From Reasoning to Verification
To further improve the performance of URSA-7B, an additional data synthesis strategy was developed that automatically generates process annotation datasets. This dataset, DualMath-1.1M, focuses on both the interpretation and the logic of mathematical solution paths. Through further training on DualMath-1.1M, URSA-7B becomes URSA-RM-7B, a verification model. URSA-RM-7B can verify the CoT solution paths generated by URSA-7B, thus significantly increasing accuracy during the testing phase. Furthermore, URSA-RM-7B shows a remarkable ability to verify out-of-distribution data, suggesting good generalizability.
Outlook and Significance for AI Research
URSA and the associated datasets MMathCoT-1M and DualMath-1.1M represent an important contribution to research in the field of multimodal mathematical reasoning. The ability to not only generate CoT reasoning but also verify it opens up new possibilities for the development of more robust and reliable AI systems. The release of the model weights, training data, and code allows the research community to build on these results and further advance the development of AI systems for mathematical reasoning.
The combination of CoT reasoning with verification mechanisms could become a new standard in the development of AI models for mathematical thinking. This is particularly relevant for applications where the correctness of the results is crucial, such as in scientific research, engineering, and finance.
Bibliographie: - https://arxiv.org/pdf/2302.00923 - https://openreview.net/forum?id=y1pPWFVfvR - https://arxiv.org/abs/2410.00151 - https://aclanthology.org/2024.findings-emnlp.268.pdf - https://www.winter-verlag.de/de/assets/download/1fxba797cb15327fcb8c54b242bc961c7b2/9783825385194/9783825385194.pdf - https://openreview.net/forum?id=KUNzEQMWU7 - https://link.springer.com/content/pdf/10.1007%2F978-1-4020-4746-6.pdf - https://www.researchgate.net/profile/Adam-Khraisat/publication/372023983_Graduate_Students'_Work_Readiness_in_the_Context_of_COVID-19_Challenges_and_Recommendations/links/65adff9fbf5b00662e334b66/Graduate-Students-Work-Readiness-in-the-Context-of-COVID-19-Challenges-and-Recommendations.pdf - https://paperswithcode.com/task/mathematical-reasoning/codeless?page=7&q= - https://papers.nips.cc/paper_files/paper/2022/hash/11332b6b6cf4485b84afadb1352d3a9a-Abstract-Conference.html