TinyLLaVA-Video-R1: A Smaller AI Model for Video Reasoning
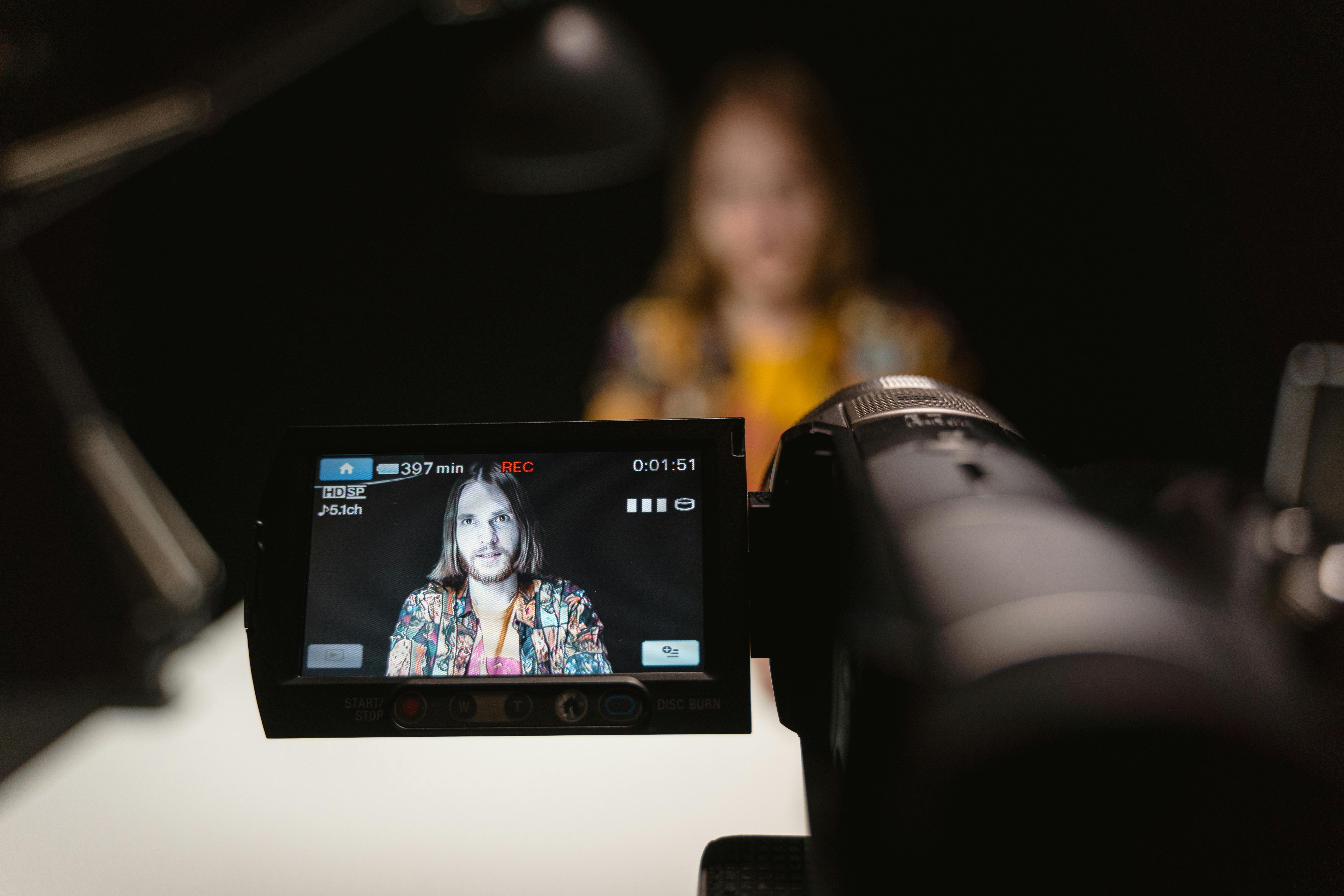
Top post
Smaller, More Powerful AI Models for Video Understanding: TinyLLaVA-Video-R1
The rapid development in the field of Artificial Intelligence (AI) is leading to increasingly powerful models that can master complex tasks such as reasoning and understanding. A focus of research is on large multimodal models (LMMs) that can process different data types like text and images. Improving their capabilities through Reinforcement Learning has recently made great strides, particularly in computationally intensive tasks such as mathematics and code generation. However, the focus on large models limits access for researchers with limited computational resources. At the same time, the explainability of AI decisions, especially in the context of general questions, is an important field of research.
Against this backdrop, TinyLLaVA-Video-R1 presents itself as a smaller AI model for video understanding, specializing in reasoning. Based on TinyLLaVA-Video, a transparently trained model with fewer than 4 billion parameters, TinyLLaVA-Video-R1 demonstrates improved reasoning and inference capabilities. The model was trained using Reinforcement Learning on general video question-answering datasets. Remarkable is the emergent ability of "aha moments," which suggests a deeper understanding of the video data.
In contrast to many existing works that focus on specialized datasets, TinyLLaVA-Video-R1 focuses on general video understanding tasks. This allows for broader applicability and opens new possibilities for research. The smaller size of the model compared to common LMMs also makes it accessible to a larger number of researchers.
The developers of TinyLLaVA-Video-R1 share their experimental results and thus offer valuable insights for future research in the field of video reasoning with smaller models. The findings should contribute to improving the efficiency and accessibility of AI models for video understanding while promoting the explainability of their decisions.
Deeper Insights into the Functionality
TinyLLaVA-Video-R1 builds on the foundation of TinyLLaVA-Video, a model already known for its transparency in the training process. By applying Reinforcement Learning, the model's ability to draw logical conclusions from video data and answer questions about it is improved. The "aha moments" represent a particularly interesting phenomenon. They indicate that the model is able to recognize connections and draw conclusions that go beyond simply reproducing information.
The research results on TinyLLaVA-Video-R1 underscore the potential of smaller AI models for complex tasks such as video understanding. Through the combination of efficient design and targeted training using Reinforcement Learning, even models with limited size can achieve impressive performance. This opens new perspectives for the development of cost-effective and accessible AI solutions.
Future Research and Application Possibilities
The development of TinyLLaVA-Video-R1 is an important step towards more efficient and accessible AI models for video understanding. The research results offer valuable insights for future development and open up diverse application possibilities. From automated video analysis to interactive learning applications – smaller, powerful models like TinyLLaVA-Video-R1 could fundamentally change the way we interact with video data.
Bibliography: Zhang, X., Wen, S., Wu, W., & Huang, L. (2025). TinyLLaVA-Video-R1: Towards Smaller LMMs for Video Reasoning. *arXiv preprint arXiv:2504.09641*. Wu, W. (2024). *Multimodal learning for video understanding*. (Doctoral dissertation, University of Sydney).