Latent Diffusion Autoencoders Show Promise for Medical Image Analysis
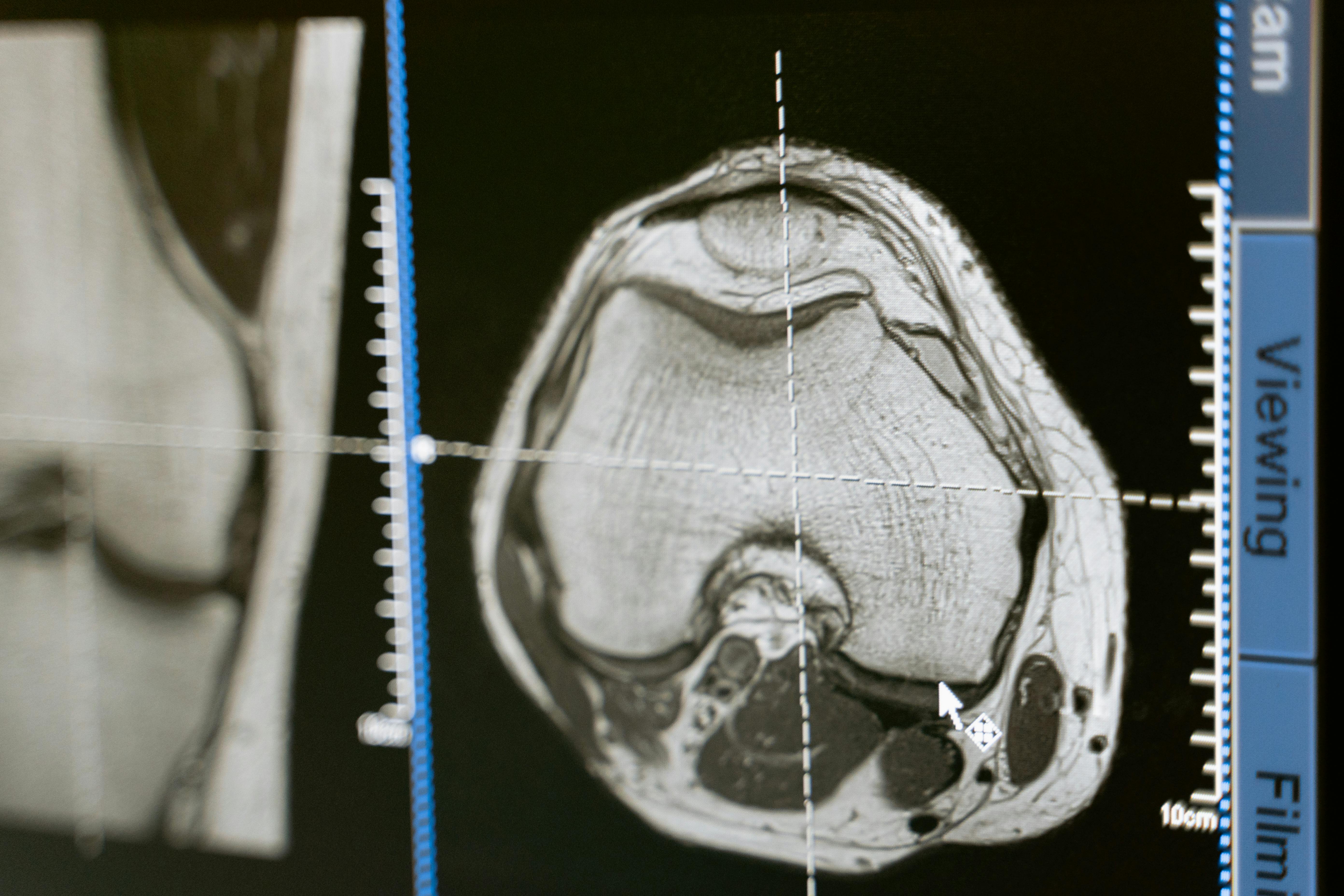
Top post
Latent Diffusion Autoencoders: A Promising Approach for Medical Image Analysis
Medical imaging plays a crucial role in the diagnosis and treatment of various diseases. Artificial intelligence (AI) has the potential to revolutionize the analysis of medical images by increasing efficiency and enabling new insights. A promising approach in this area is so-called Latent Diffusion Autoencoders (LDAEs). These novel frameworks leverage the power of diffusion models to learn meaningful representations of medical image data.
Functionality and Advantages of LDAEs
Traditional autoencoders compress image data into a latent space and then reconstruct it. LDAEs extend this principle by applying the diffusion process in the latent space. This leads to significantly higher efficiency, especially when processing three-dimensional image data such as MRI scans of the brain. By applying the diffusion process in the compressed latent space, the computational effort is significantly reduced, which enables the analysis of large datasets and makes the application of LDAEs for 3D imaging practicable.
Another advantage of LDAEs lies in their ability to learn semantically meaningful representations. This means that the learned latent vectors contain information about relevant features of the image data, such as anatomical structures or disease characteristics. These semantic representations can be used for various tasks, including disease classification, disease progression prediction, and the generation of synthetic image data.
Application of LDAEs in Alzheimer's Research
A recent study investigated the application of LDAEs to MRI scans of the brains of patients with Alzheimer's disease. The results show that LDAEs are able to learn meaningful representations that correlate with age and the progression of Alzheimer's disease. The learned representations enabled accurate diagnosis of Alzheimer's (ROC-AUC: 90%, accuracy: 84%) and accurate age estimation (MAE: 4.1 years, RMSE: 5.2 years).
Furthermore, the study demonstrated the ability of LDAEs for semantic manipulation of image data. This makes it possible to make targeted changes to the image data, for example, to investigate the influence of certain anatomical features on the diagnosis. Semantic interpolation, i.e., the generation of intermediate images between two given images, also showed promising results. Even with larger time intervals between the images, the model was able to realistically reconstruct the changes in the brain, suggesting the ability to capture temporal trends.
Future Perspectives and Potentials
LDAEs offer enormous potential for medical image analysis. The combination of high efficiency and the ability to learn semantically meaningful representations opens up new possibilities for the diagnosis, treatment, and research of diseases. Future research could focus on the application of LDAEs in other medical imaging areas, such as oncology or cardiology. The development of new methods for semantic manipulation and the improvement of the interpretability of the learned representations are also important research goals.
The development of LDAEs is an important step towards more efficient and precise medical image analysis. This technology has the potential to improve healthcare and gain new insights into complex disease processes.
Bibliography: https://arxiv.org/abs/2504.08635 https://openaccess.thecvf.com/content/CVPR2022/papers/Preechakul_Diffusion_Autoencoders_Toward_a_Meaningful_and_Decodable_Representation_CVPR_2022_paper.pdf https://chatpaper.com/chatpaper/?id=4&date=1744560000&page=1 https://www.researchgate.net/publication/363908515_Diffusion_Autoencoders_Toward_a_Meaningful_and_Decodable_Representation https://generative-vision.github.io/workshop-CVPR-24/papers/59.pdf https://ar5iv.labs.arxiv.org/html/2111.15640 https://openaccess.thecvf.com/content/CVPR2022/html/Preechakul_Diffusion_Autoencoders_Toward_a_Meaningful_and_Decodable_Representation_CVPR_2022_paper.html https://proceedings.mlr.press/v202/wang23ah/wang23ah.pdf https://www.researchgate.net/publication/348146131_Recent_Advances_in_Variational_Autoencoders_With_Representation_Learning_for_Biomedical_Informatics_A_Survey https://www.semanticscholar.org/paper/Unsupervised-Representation-Learning-from-Diffusion-Zhang-Zhao/57655bdf9bfaf326ca73e5843edeb3e9f2c4d315