New AI Method Completes Missing Data in LiDAR Scans
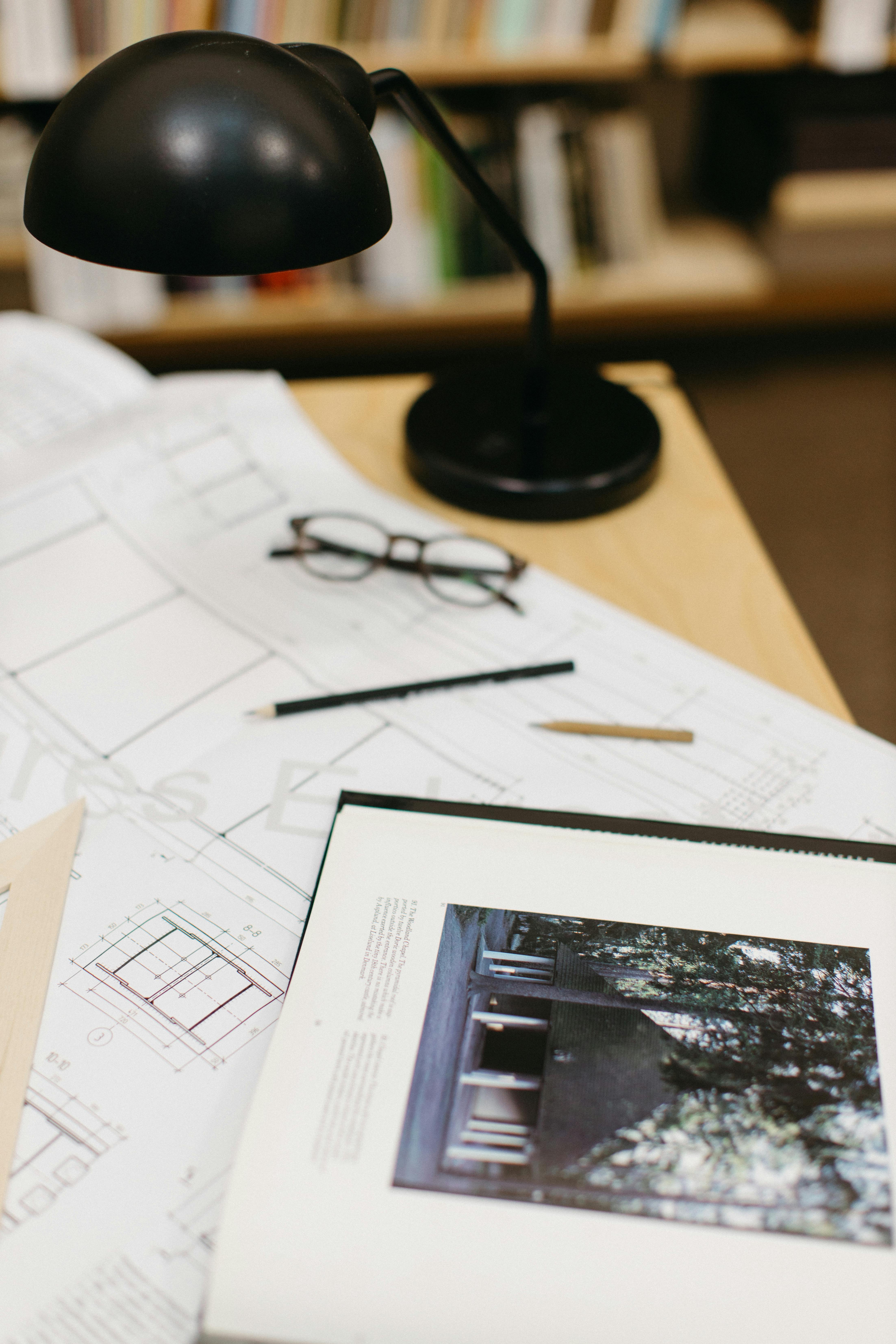
Top post
Seamless 3D Perception: New AI Method Completes LIDAR Scans
Environmental perception is a central challenge for autonomous systems. While LIDAR sensors provide precise 3D point clouds, these are often incomplete and contain gaps. A new method called CAL (Complete Anything in Lidar) promises to close these gaps and thus enable a more complete image of the environment. Developed by researchers at NVIDIA, CAL uses a novel approach to complete and recognize objects in LIDAR scans, even if they are only partially captured.
Conventional methods for completing LIDAR data often rely on a fixed vocabulary of object classes. Therefore, they can only recognize and complete objects that were already present in the training data. CAL, on the other hand, pursues a zero-shot approach. This means the system can also recognize and complete objects that it has never "seen" before. This is achieved through the use of multimodal sensor data and temporal context. By analyzing image sequences in which objects are captured from different perspectives and at different times, CAL learns to extract the shape and semantic features of objects.
This learning process is based on the distillation of knowledge from multimodal data into a purely LIDAR-based model. Although the system initially only learns partial shapes of objects, it is able to infer complete object shapes by combining multiple partial observations in the dataset. The result is a model that not only completes objects, but also localizes them as amodal 3D bounding boxes and recognizes them beyond fixed class vocabularies. This opens up new possibilities for applications in areas such as autonomous driving, robotics, and 3D modeling.
How CAL Works: A Look Behind the Scenes
CAL uses the temporal information from multimodal sensor data to develop an understanding of the shape and semantic properties of objects. This information is then distilled into a specialized model trained purely on LIDAR data. The model learns to complete incomplete LIDAR scans and recognize objects by applying the learned shapes and features. A particular advantage of CAL is its ability to recognize and complete objects that were not included in the training dataset. This is made possible by the zero-shot approach, which is based on the generalization of learned knowledge.
Applications and Potential
The ability to complete incomplete LIDAR data and robustly recognize objects is crucial for a variety of applications. In the field of autonomous driving, CAL can help improve the safety and reliability of vehicles by providing a more complete picture of the environment. In robotics, the technology can enable robots to grasp and manipulate objects more precisely. CAL also offers new possibilities in 3D modeling and reconstruction to create detailed and realistic models of environments. Research on CAL and similar methods contributes to pushing the boundaries of 3D perception and opening up new application areas for AI-based systems.
Future Perspectives
The development of CAL represents an important step towards more robust and flexible 3D object recognition and completion. Future research could focus on improving the accuracy and efficiency of the model, as well as expanding the application areas. The combination of CAL with other AI methods, such as semantic segmentation, could lead to even more powerful systems that enable a comprehensive understanding of the environment. The development of specialized hardware solutions could further improve the real-time capability of CAL and enable its use in demanding applications such as autonomous driving.
Bibliography: Takmaz, A., Saltori, C., Peri, N., Meinhardt, T., de Lutio, R., Leal-Taixé, L., & Ošep, A. (2025). Towards Learning to Complete Anything in Lidar. *arXiv preprint arXiv:2504.12264*. Chen, D. Z., Han, S., Wang, B., & Liu, Y. (2024). Learning Compact Representations for LiDAR Completion and Generation. *arXiv preprint arXiv:2311.01448*. Guo, Y., Li, J., Zhang, F., Liang, X., Xiao, J., & Wang, R. (2024). LiDAR-Net: A Real-scanned 3D Point Cloud Dataset for Indoor Scenes. *Proceedings of the IEEE/CVF Conference on Computer Vision and Pattern Recognition*, 11059-11069. Milioto, A., Vizzo, I., Behley, J., & Stachniss, C. (2024). Panoptic Segmentation for Large-Scale Point Clouds. *arXiv preprint arXiv:2403.13129*. Qiu, Z., Jaritz, M., Gu, J., Zhou, Y., Sun, L., Dai, A., ... & Yu, F. (2024). Towards learning to complete anything in lidar. *ECCV*.