Multi-Image Grounding Advances Multimodal Large Language Models
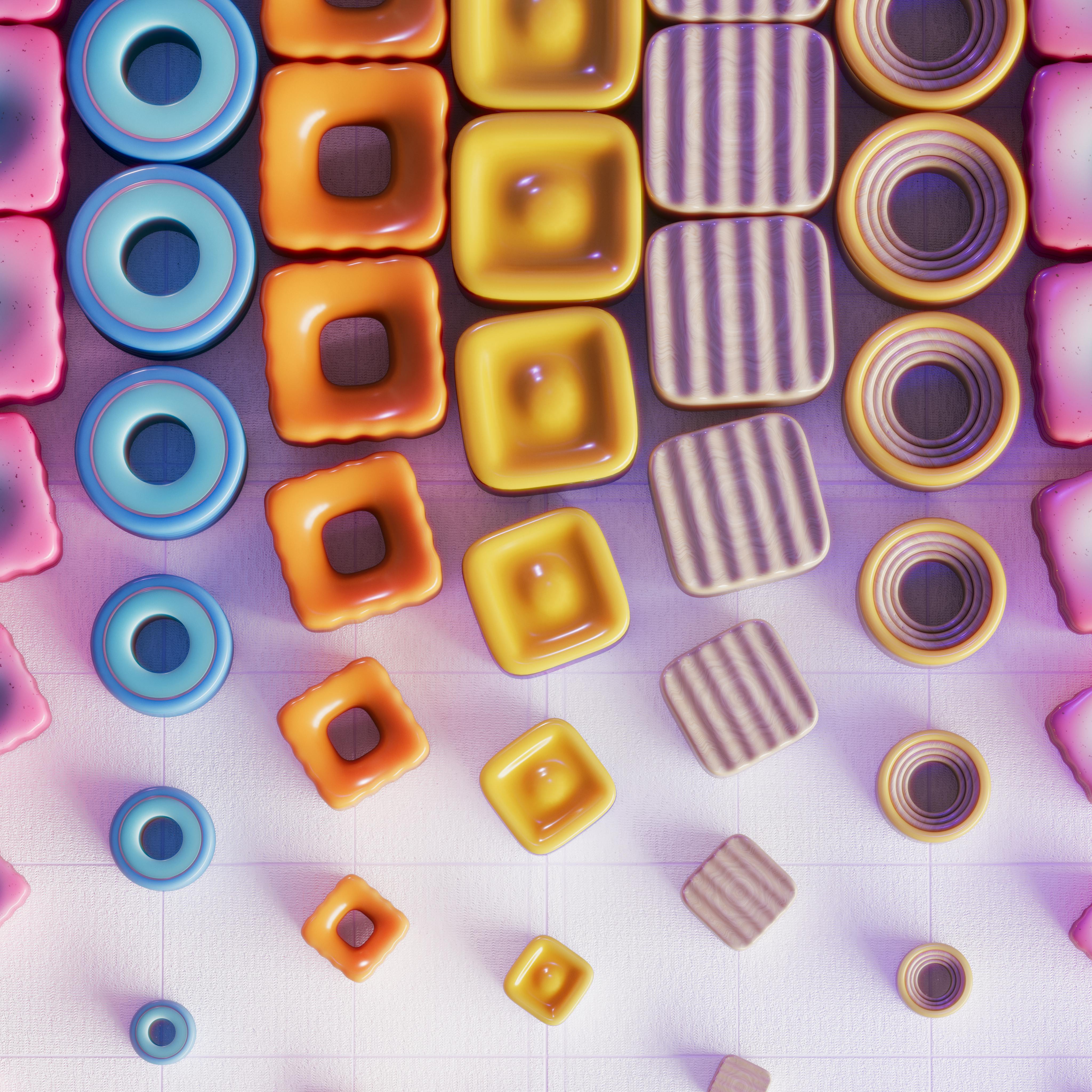
Top post
Multimodal Large Language Models (MLLMs) have made impressive progress in recent years in processing and interpreting image and text data. They can not only analyze individual images in detail, but also recognize relationships between multiple images. Despite this progress, challenges remain, particularly in the precise localization of objects or areas within complex multi-image scenarios. This article highlights the latest developments in Multi-Image Grounding (MIG) and introduces Migician, a model that addresses these challenges.
The Challenge of Multi-Image Grounding
Previous MLLMs have focused primarily on so-called Single-Image Grounding, i.e., the localization of objects within a single image. However, the precise linking of text descriptions with visual elements across multiple images – Multi-Image Grounding – represents a significantly more complex task. Here, the model must not only understand the individual images but also grasp the relationships and context between them. An example of this would be searching for a specific object in a series of images, based on a complex textual query that refers to several visual features and their relationships.
A First Approach: Chain-of-Thought (CoT)
An initial approach to solving the MIG problem is the application of the Chain-of-Thought (CoT) framework. This framework combines the capabilities of MLLMs in Single-Image Grounding with their understanding of multi-image relationships. In the first step, the model generates a textual description of the searched object based on the multi-image information. In the second step, it uses this description to locate the object within the images. Although this approach is promising in simple scenarios, it shows weaknesses in describing abstract visual information and requires significantly more computation time due to the two-stage process.
Migician: A New Standard for Multi-Image Grounding
To overcome the limitations of the CoT approach, Migician was developed. This model enables direct, precise, and free-form grounding across multiple images. Migician was trained with a two-stage training process based on the newly created MGrounding-630k dataset. This dataset contains a variety of MIG tasks, derived from existing datasets, as well as newly generated data for free-form grounding. In the first training step, the general grounding ability of the model is improved. In the second step, Migician is specifically trained on free-form MIG.
MIG-Bench: A Benchmark for Multi-Image Grounding
To evaluate the performance of Migician and other MLLMs in the field of MIG, MIG-Bench was developed. This comprehensive benchmark comprises ten different tasks with a total of 5,900 images and over 4,200 test instances. The results show that Migician significantly outperforms existing MLLMs in Multi-Image Grounding and even surpasses larger models with 70 billion parameters. This highlights the potential of specialized models like Migician for complex multi-image scenarios.
The Future of Multi-Image Grounding
Migician and MIG-Bench represent an important step in the development of MLLMs. They open up new possibilities for applications in areas such as autonomous driving, surveillance systems, and robotics. The ability to precisely and flexibly locate objects in complex multi-image scenarios is essential for the development of intelligent systems that are capable of interpreting the visual world similarly to humans. Future research will focus on further improving the performance of MIG models and exploring new fields of application.
```