RealSyn: A New Paradigm for Vision-Language Representation Learning from Multimodal Interleaved Documents
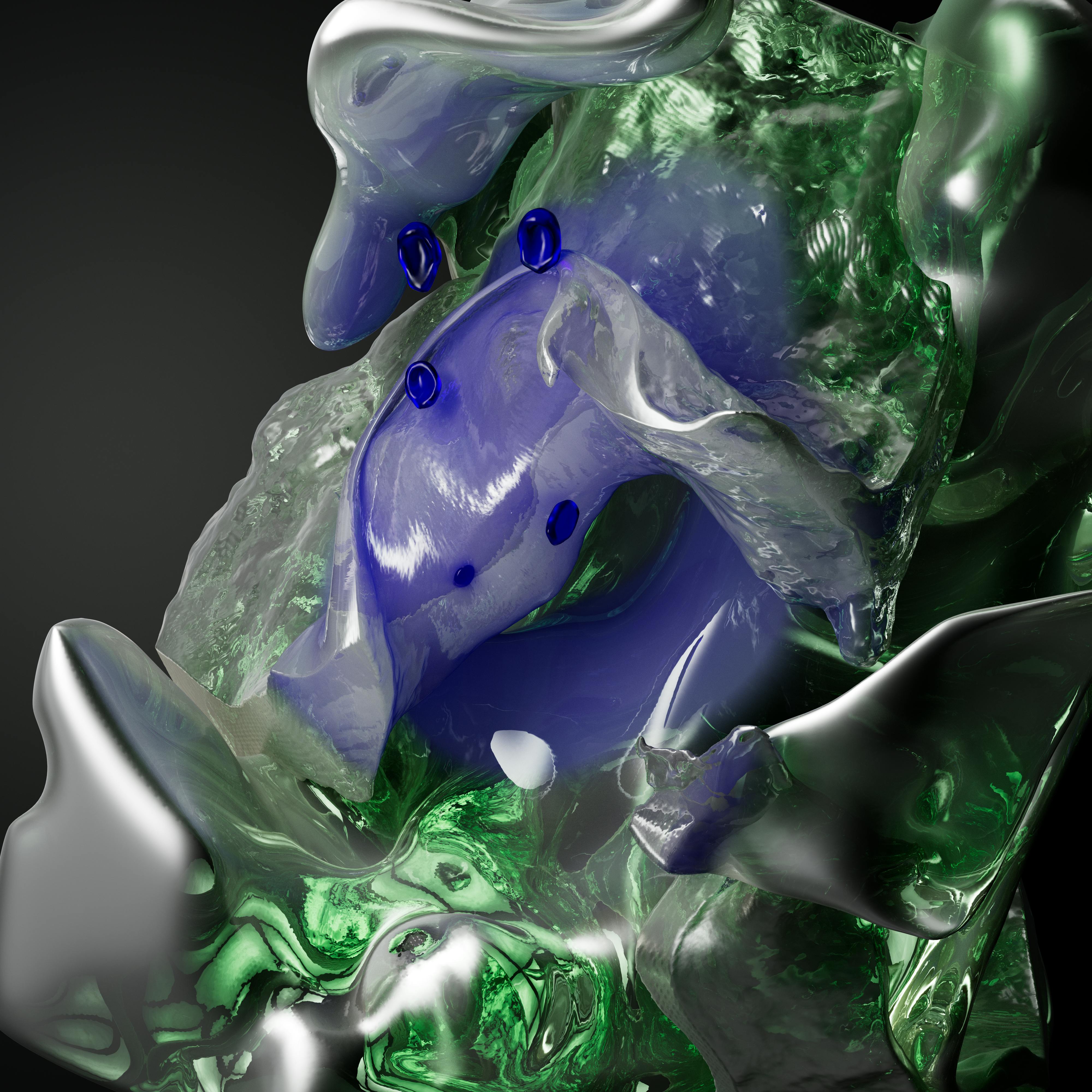
Top post
RealSyn: A New Approach for Multimodal Interleaved Documents in the Vision-Language Domain
The combination of image and text data has proven to be a crucial factor for advancements in Artificial Intelligence in recent years. Models like CLIP (Contrastive Language-Image Pre-training) have achieved impressive results in various benchmarks by training with extensive image-text pairs. However, a large amount of untapped potential remains in the form of multimodal interleaved documents, which contain both images and text, but are not explicitly linked in pairs. A new paradigm, RealSyn, addresses this challenge and enables the utilization of this data for vision-language representation learning.
RealSyn is based on the idea of effectively extracting and linking the information contained in multimodal interleaved documents. A multi-stage pipeline has been developed for this purpose. First, high-quality images and texts are extracted from the documents using a "Real-World Data Extraction Pipeline." Subsequently, a hierarchical retrieval method ensures that each image is linked with several semantically relevant texts. This step is crucial for understanding the context of the images and improving the representation quality.
To further enhance the understanding of fine-grained visual information, RealSyn utilizes a module for generating synthetic texts, enriched with visual semantic information. These synthetic texts complement the realistic texts and provide additional training data for the model. Another important aspect of RealSyn is the use of a semantic balance sampling strategy. This strategy ensures that the model is also trained with concepts that occur less frequently in the data (long-tail concepts), thereby improving the model's generalization ability.
The result of these innovations is the RealSyn dataset, which combines realistic and synthetic texts and is available in three sizes: 15 million, 30 million, and 100 million datasets. This scalability allows the dataset to be adapted to the needs of various research and application areas.
Extensive experiments have shown that RealSyn effectively advances vision-language representation learning and exhibits excellent scalability. Models trained with RealSyn achieve state-of-the-art performance in various downstream tasks. This underscores the potential of RealSyn to further drive development in the field of Artificial Intelligence.
The publication of the RealSyn dataset and the pre-trained model weights on platforms like GitHub allows the research community to build on these advances and develop new applications in the field of vision-language learning. For companies like Mindverse, which specialize in the development of AI solutions, RealSyn offers new opportunities to improve existing products and develop innovative applications in areas such as chatbots, voicebots, AI search engines, and knowledge systems.
The ability to effectively utilize multimodal interleaved documents opens up new perspectives for understanding and processing information. RealSyn represents an important step in this direction and contributes to exploiting the full potential of multimodal data.
Bibliographie: - https://huggingface.co/datasets/Kaichengalex/RealSyn15M - arxiv:2502.12513 - RealSyn: An Effective and Scalable Multimodal Interleaved Document Transformation Paradigm