Sa2VA: A New Model for Dense Grounded Understanding of Images and Videos
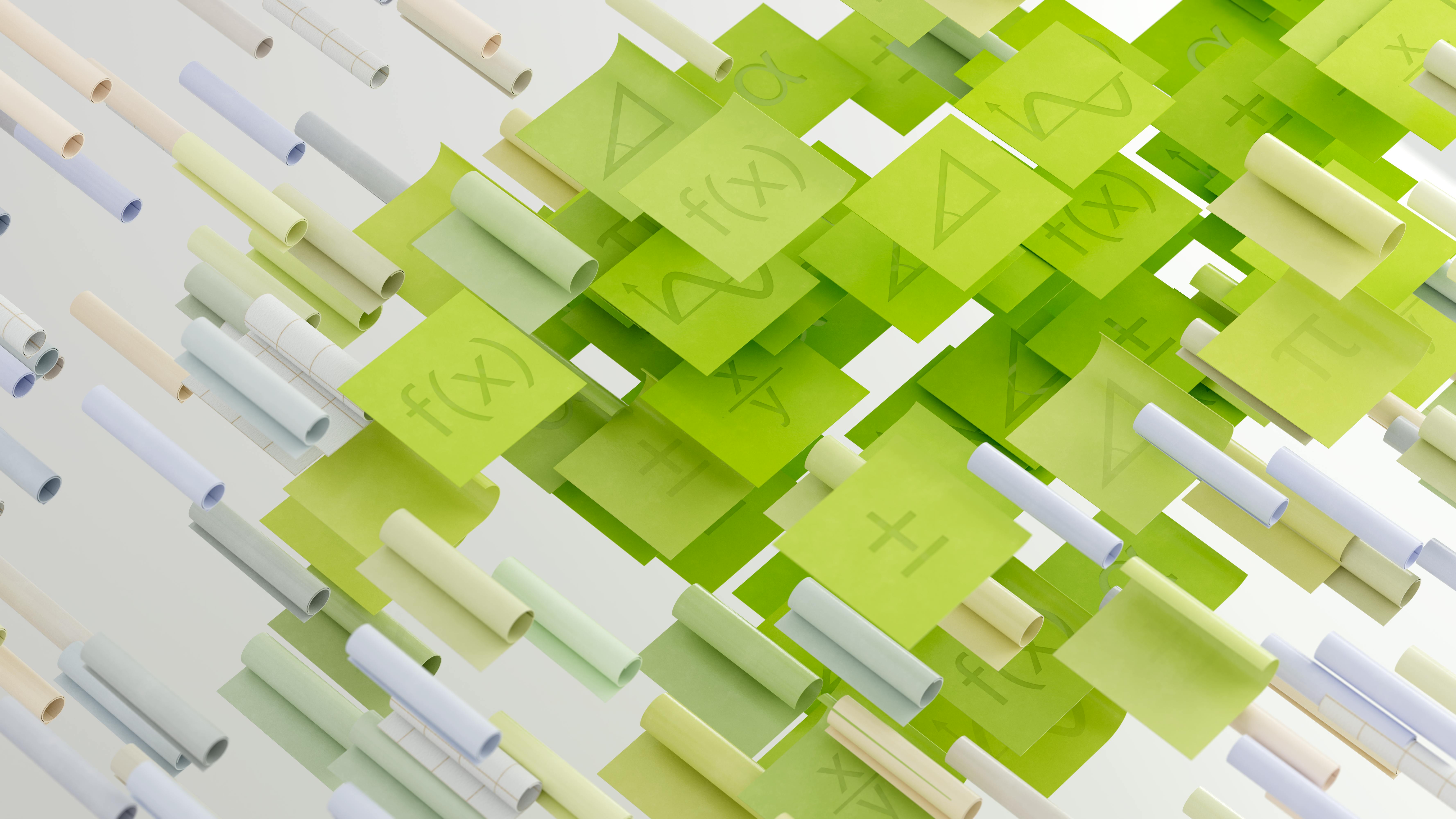
Top post
Sa2VA: A New Approach for Dense, Grounded Understanding of Images and Videos
The rapid development in the field of multimodal large language models (MLLMs) continuously opens up new possibilities in understanding and interacting with visual content. Sa2VA, a novel model, promises significant progress in the dense, grounded understanding of images and videos. In contrast to previous MLLMs, which are often limited to specific modalities and tasks, Sa2VA supports a wide range of image and video tasks, including referring segmentation and conversation, with minimal one-shot instruction tuning.
Merging Two Worlds: SAM-2 and LLaVA
Sa2VA combines the strengths of two established models: SAM-2, a foundational video segmentation model, and LLaVA, an advanced vision-language model. By unifying text, image, and video in a shared LLM token space, Sa2VA generates so-called instruction tokens. These instructions guide SAM-2 in creating precise masks, enabling a grounded, multimodal understanding of both static and dynamic visual content.
Ref-SAV: A New Dataset for Referring Video Segmentation
To enhance the model's performance, Ref-SAV was developed, an automatically labeled dataset with over 72,000 object expressions in complex video scenes. Existing datasets for referring video segmentation are often limited to small datasets and short clips with little overlap. Ref-SAV addresses these limitations and provides a foundation for the development and evaluation of more robust models. Additionally, 2,000 video objects in Ref-SAV were manually validated to create a benchmark for referring video object segmentation in complex environments.
Diverse Applications Through One-Shot Instruction Tuning
Sa2VA supports various tasks in the one-shot instruction tuning format, including:
Referring Segmentation: Identifying and segmenting objects based on textual descriptions.
Visual Question Answering (VQA): Answering questions about visual content.
Grounded Conversational Generation (GCG): Conducting natural language conversations based on visual content.
These capabilities open up potential for complex real-world applications, such as short video editing, robot navigation, and surveillance analysis. Through prompt-based, fine-grained analysis of videos, users can interact with the video material in real-time and make targeted queries.
A Scalable and Future-Proof Design
Sa2VA is characterized by a scalable and future-proof design. End-to-end training enables efficient use of large datasets and the integration of new advancements in the field of MLLMs. By freezing the decoder and memory module of SAM-2, the perception and tracking capabilities are preserved, and the model can be updated as a plug-and-play module with the latest MLLMs.
Sa2VA and Mindverse: A Strong Team for AI-Powered Content Creation
The developments surrounding Sa2VA are particularly relevant for companies like Mindverse, which offer AI-powered content solutions. The ability to semantically understand images and videos and interact with them on a fine-grained level opens up new possibilities for automated content creation, analysis, and editing. From generating image captions to creating complex video scenarios, Sa2VA could be a key building block for the next generation of AI-powered content tools.
Bibliography Yuan, H., Li, X., Zhang, T., Huang, Z., Xu, S., Ji, S., Tong, Y., Qi, L., Feng, J., & Yang, M.-H. (2025). Sa2VA: Marrying SAM2 with LLaVA for Dense Grounded Understanding of Images and Videos. arXiv preprint arXiv:2501.04001. Yuan, H., Li, X., Zhang, T., Huang, Z., Xu, S., Ji, S., Tong, Y., Qi, L., Feng, J., & Yang, M.-H. (2025). Sa2VA: Marrying SAM2 with LLaVA for Dense Grounded Understanding of Images and Videos. arXiv preprint arXiv:2501.04001v1. ByteDance. Sa2VA-4B. Hugging Face. https://huggingface.co/ByteDance/Sa2VA-4B Hugging Face. Papers. https://huggingface.co/papers ChatPaper. Sa2VA: Marrying SAM2 with LLaVA for Dense Grounded Understanding of Images and Videos. https://chatpaper.com/chatpaper/ja?id=4&date=1736265600&page=1