Masked Scene Modeling Advances Self-Supervised 3D Scene Understanding
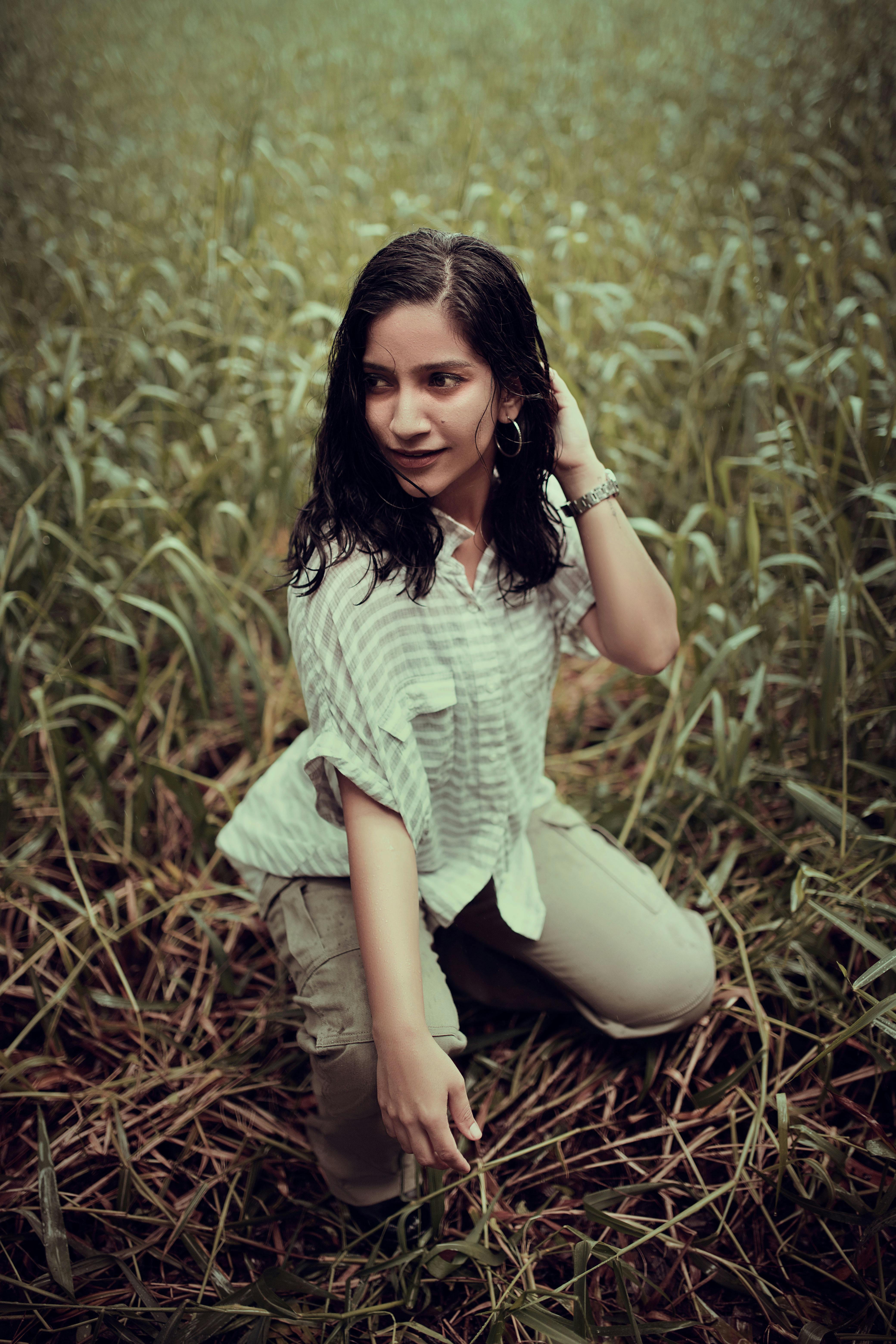
Top post
Self-Supervised Learning in 3D Scene Understanding: New Advances through Masked Scene Modeling
Understanding 3D scenes is a central challenge in Artificial Intelligence, with applications in areas such as robotics, autonomous driving, and augmented reality. While supervised learning has achieved impressive results in the past, it requires large amounts of annotated data, which is time-consuming and expensive to create. Self-supervised learning offers a promising alternative by allowing models to learn from unannotated data. So far, however, self-supervised methods in 3D scene understanding have mainly been used for weight initialization before fine-tuning for specific tasks. This limits their application as a source of general, versatile features.
Recent research now shows a promising way to close this gap between supervised and self-supervised learning. A new approach, known as "Masked Scene Modeling" (MSM), allows the training of self-supervised models that extract features comparable in quality to those of supervised models. MSM is based on the idea of masking parts of a 3D scene and training the model to reconstruct the missing information. This approach forces the model to develop a deep understanding of the scene without relying on explicit labels.
A significant contribution of this research lies in the development of a robust evaluation protocol for self-supervised features in 3D scene understanding. This protocol utilizes hierarchical models and multi-resolution feature sampling to generate meaningful, point-based representations. These representations capture the semantic capabilities of the model and are suitable for evaluation using linear tests and nearest-neighbor methods.
The results show that models trained with MSM are competitive with supervised models in direct comparison when using exclusively pre-trained features in a linear setup. Furthermore, MSM significantly outperforms existing self-supervised approaches. The success of MSM is attributed in particular to the native 3D-based training method and the bottom-up approach to reconstructing masked areas. This approach is specifically tailored to hierarchical 3D models and allows the model to develop a comprehensive understanding of the spatial relationships within the scene.
These developments open up new possibilities for the use of self-supervised learning in 3D scene understanding. The availability of powerful, pre-trained models could accelerate the development of new applications in various fields and reduce the need for large, annotated datasets. Future research could focus on further improving the MSM method, as well as exploring new application areas for the generated features.
For companies like Mindverse, which specialize in AI-based content creation, chatbots, voicebots, and knowledge databases, these advances in 3D scene understanding are particularly relevant. The improved ability to understand and process 3D data could lead to innovative applications in areas such as virtual product design, interactive 3D environments, and personalized user experiences.
Bibliography: - Jiang et al. Self-Supervised Pre-Training With Masked Shape Prediction for 3D Scene Understanding. CVPR 2023. - Hermosilla, P., Stippel, C., & Sick, L. Masked Scene Modeling: Narrowing the Gap Between Supervised and Self-Supervised Learning in 3D Scene Understanding. arXiv preprint arXiv:2504.06719 (2025). - Li, Y. et al. (Title not available). NeurIPS 2023. - (Further source without title) ResearchGate. 3D Human Shape and Pose from a Single Low-Resolution Image with Self-Supervised Learning.