AI Models Enhance Research on Diachronic Linguistic Change
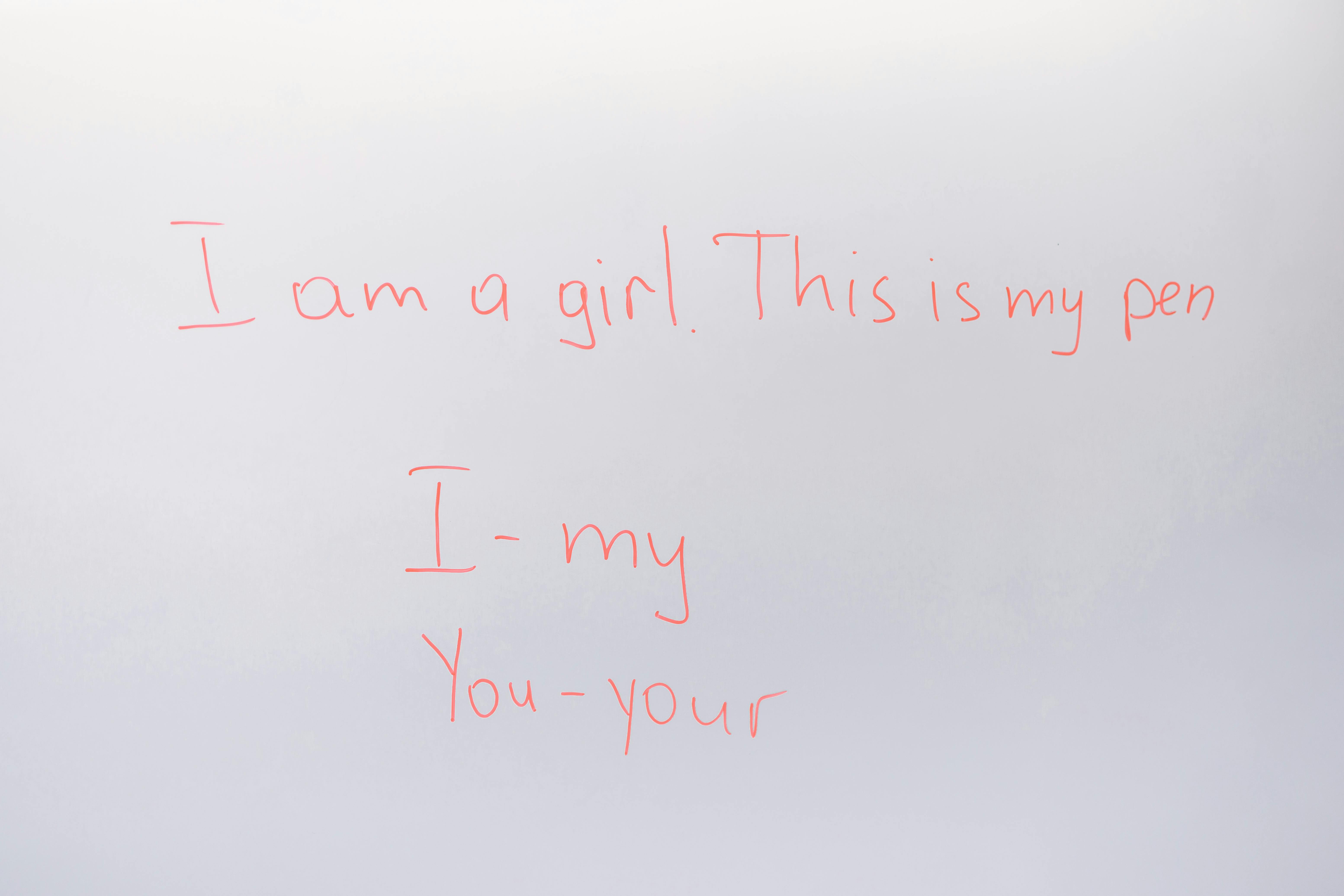
Top post
Focus on Language Change: AI Models for Research in Diachronic Linguistics
Large language models (LLMs) are increasingly being used in scientific research, including in the humanities. Especially in historical linguistics and literary studies, where arguments often rely on classifications such as genre or time period, LLMs offer new possibilities. While existing approaches attempt to constrain inference by fine-tuning or model editing to specific domains, researchers argue that domain-specific pretraining represents the most reliable method. However, this is often associated with high data and computational costs.
A recent study investigates how efficient pretraining techniques can generate useful models for corpora that are too large for manual review, but too small for typical LLM approaches. The researchers developed a novel date-mapping pipeline to create a temporally segmented dataset of five 10-million-word partitions. Based on this, they trained two corresponding five-model batteries: one with efficient pretraining and one with fine-tuned Llama3-8B parameters.
The results show that the pretrained models are faster to train than the fine-tuned base models and better reflect the historical divisions of the corpus. Prioritizing speed and precision over ahistorical completeness enables new approaches to hypothesis generation and testing in the target domains. Using diachronic linguistics as an example, the researchers demonstrate that their method allows for the detection of various phenomena, including lexical changes, non-lexical (grammatical and morphological) changes, as well as the introduction and obsolescence of word meanings.
Efficient Pretraining as Key
The study highlights the importance of efficient pretraining. In contrast to resource-intensive methods like LoRA (Low-Rank Adaptation), pretraining enables a more cost-effective investigation of language change phenomena. The researchers emphasize that targeted pretraining on specific time periods allows for a deeper understanding of linguistic development. This opens new avenues for researching changes in grammar, the emergence of new word meanings, and other linguistic phenomena.
Applications and Future Research
The presented date-mapping and model training pipeline provides a promising foundation for future research in historical linguistics and other humanities disciplines. The method can be transferred to other target areas with minimal adjustments, enabling the investigation of language change phenomena in various contexts. The researchers provide a ready-to-use pipeline that facilitates the application of the approach to other research fields.
The combination of efficient pretraining and temporally segmented datasets opens new possibilities for the study of diachronic language change. The presented method not only allows for the identification of lexical and grammatical changes but also the analysis of semantic shifts and the emergence of new word meanings. The results of the study underscore the potential of LLMs as a tool for scientific discovery in the humanities.
Bibliography: - http://arxiv.org/abs/2504.05523 - https://arxiv.org/html/2504.05523v1 - https://www.researchgate.net/publication/390601344_Pretraining_Language_Models_for_Diachronic_Linguistic_Change_Discovery - https://www.themoonlight.io/fr/review/pretraining-language-models-for-diachronic-linguistic-change-discovery - https://aclanthology.org/2024.bucc-1.2.pdf - https://www.degruyter.com/document/doi/10.1515/9783110251609.1599/html - https://web.stanford.edu/~jurafsky/pubs/paper-hist_vec.pdf - https://www.mn.uio.no/ifi/english/people/aca/andreku/shifts.pdf - https://www.sciencedirect.com/science/article/abs/pii/S030645732400284X - https://www.researchgate.net/publication/363394448_Temporal_Effects_on_Pre-trained_Models_for_Language_Processing_Tasks