Training-Free Control of Text-to-Video Generation with Multimodal Planning
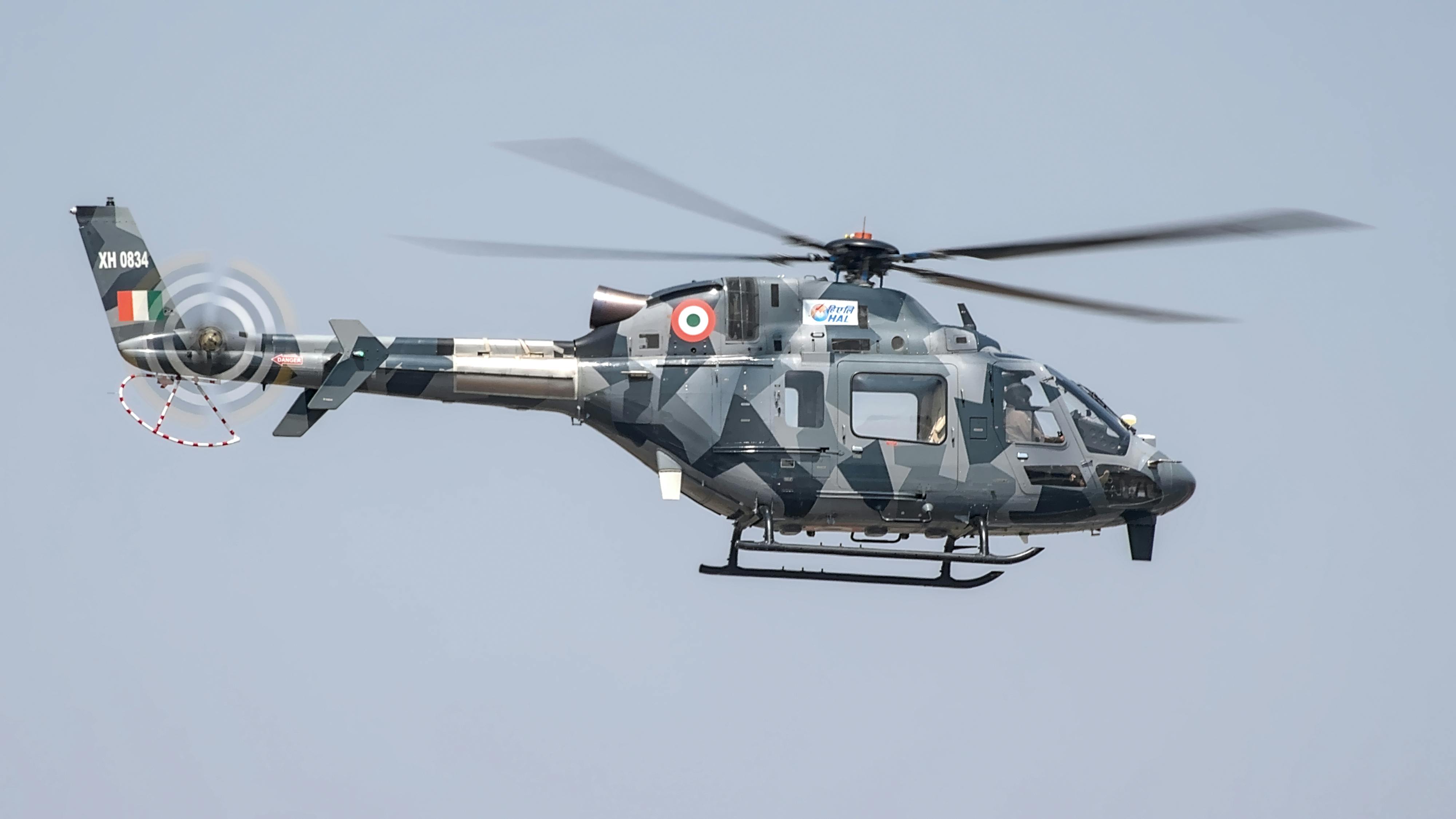
Top post
Training-Free Guidance in Text-to-Video Generation: A New Approach through Multimodal Planning and Structured Noise Initialization
The generation of videos from text descriptions has made enormous progress in recent years thanks to advanced diffusion models. The quality of the generated videos has improved significantly, but the precise implementation of complex text prompts, particularly regarding spatial arrangement and object movements, remains a challenge. Existing approaches to controlling video generation, such as layout-based methods, often require fine-tuning of the models or iterative manipulations during the generation process. This leads to increased memory requirements and makes it difficult to use large, powerful text-to-video models.
A promising new approach called Video-MSG offers a training-free control method for text-to-video generation. Video-MSG is based on multimodal planning and structured noise initialization and bypasses the disadvantages of existing methods. The process is divided into three steps. In the first two steps, Video-MSG creates a detailed spatio-temporal plan, referred to as a "Video Sketch." This Video Sketch serves as a blueprint for the final video and defines the background, foreground, and object movements in the form of draft frames. In the last step, Video-MSG controls a downstream text-to-video diffusion model using the Video Sketch through noise inversion and denoising.
A key advantage of Video-MSG is that no fine-tuning or manipulation of the attention mechanisms is required during inference. This reduces memory requirements and facilitates the use of large text-to-video models. The effectiveness of Video-MSG has been demonstrated using various text-to-video models such as VideoCrafter2 and CogVideoX-5B on established benchmarks like T2VCompBench and VBench. The results show improved consistency of the generated videos with the text descriptions.
How does Video-MSG work in detail?
The core of Video-MSG lies in the creation of the Video Sketch. This includes the planning of the spatial arrangement and the temporal sequences in the video. Background information is generated, for example, by text-to-image generators, while objects in the foreground are segmented, and their movements are planned. This information is recorded in the draft frames of the Video Sketch.
In the final step, Video-MSG uses the Video Sketch to control the diffusion model. Through noise inversion, the Video Sketch is projected into the noise space of the diffusion model. Subsequently, the diffusion model is guided by the denoised and structured input to generate the final video.
Outlook and Potential
Video-MSG opens up new possibilities for controlling text-to-video models. The training-free method simplifies the application of large and complex models. Future research could focus on improving Video Sketch generation to implement even more complex scenarios and more detailed descriptions. The combination of multimodal planning and structured noise initialization could also find application in other areas of generative AI.
Bibliographie Li, J., Yu, S., Lin, H., Cho, J., Yoon, J., & Bansal, M. (2025). Training-free Guidance in Text-to-Video Generation via Multimodal Planning and Structured Noise Initialization. *arXiv preprint arXiv:2504.08641*. arxiv.org/abs/2504.08641 arxiv.org/html/2504.08641v1 paperreading.club/page?id=298902 chatpaper.com/chatpaper/?id=4&date=1744560000&page=1 www.ecva.net/papers/eccv_2024/papers_ECCV/papers/06012.pdf www.researchgate.net/publication/389821108_VideoMerge_Towards_Training-free_Long_Video_Generation proceedings.neurips.cc/paper_files/paper/2024/file/3cbf33008024aa1612ce853ef78e0e53-Paper-Conference.pdf github.com/soraw-ai/Awesome-Text-to-Video-Generation/blob/main/README.md github.com/showlab/Awesome-Video-Diffusion proceedings.neurips.cc/paper_files/paper/2024/file/345208bdbbb6104616311dfc1d093fe7-Paper-Conference.pdf