AI-Powered MangaNinja Enables Precise Colorization of Line Art
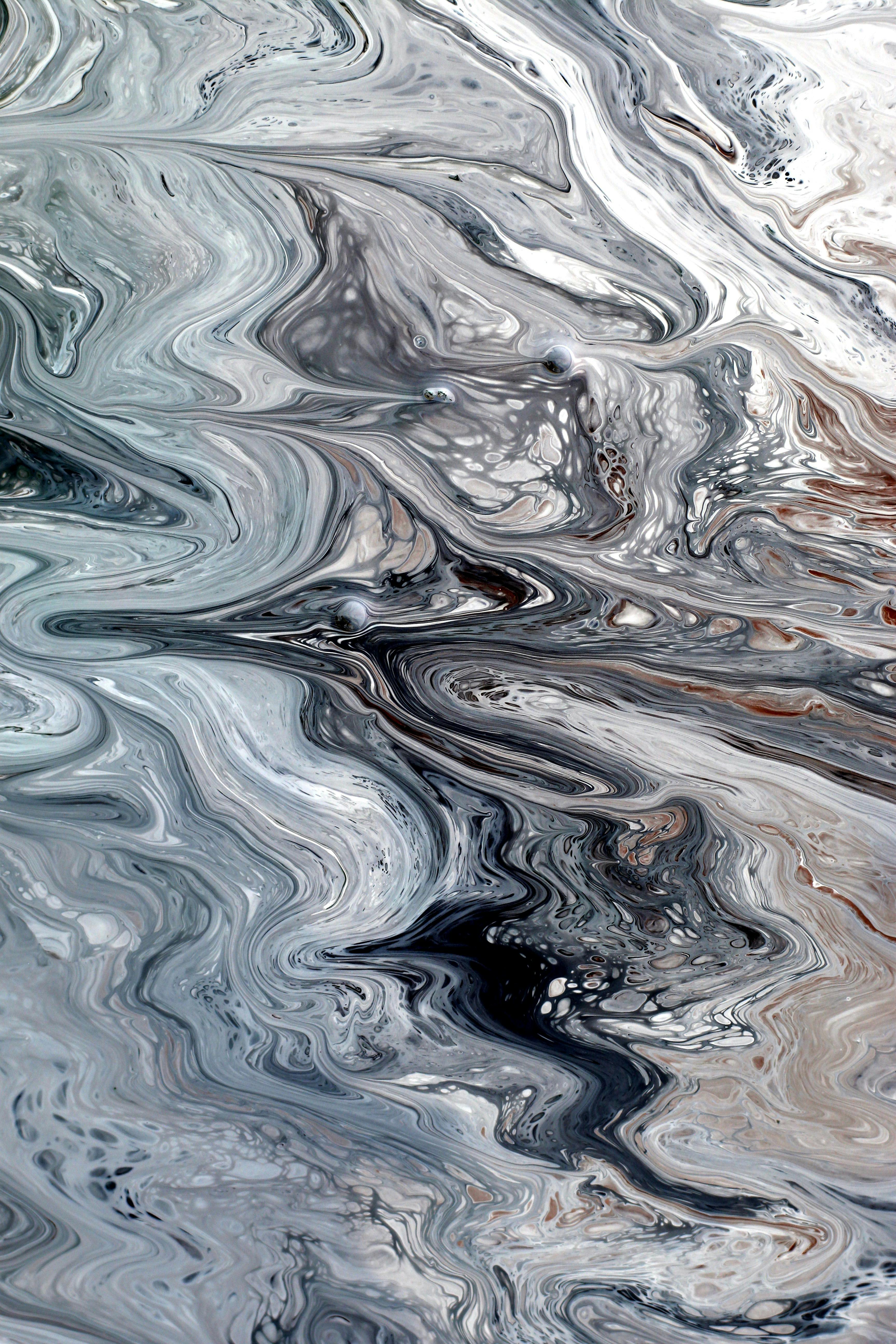
Top post
From Lines to Vivid Colors: AI-Powered Colorization of Line Art with MangaNinja
The world of artificial intelligence (AI) is constantly revolutionizing creative processes. One particularly exciting field is the automatic colorization of line art, which opens up new possibilities for artists and designers. MangaNinja, a method based on diffusion models, presents an innovative approach to this task, characterized by precise color transfer from reference images.
Precision through Innovative Mechanisms
MangaNinja relies on two central mechanisms to ensure the detailed transfer of colors from reference images to line art. First, it uses a "Patch Shuffling" module. This module promotes the learning of correspondences between the reference color image and the line art to be colorized. By selectively mixing image patches, the model learns which color areas in the reference image belong to which line segments in the line art. This allows for more precise color assignment compared to conventional methods.
The second key mechanism is point-controlled control. This allows for fine-tuned color adjustment. By placing points on the line art and assigning corresponding colors from the reference image, the user can specifically influence the colorization process. This interactive control allows even complex scenarios and difficult cases to be mastered, which posed a challenge for previous algorithms.
Superior Performance Compared to Existing Solutions
The developers of MangaNinja have demonstrated the performance of their model using a custom-built benchmark. The results show that MangaNinja achieves significantly higher precision in colorization compared to current solutions. In particular, the ability to transfer colors faithfully from reference images distinguishes MangaNinja from other methods.
Versatile Applications
The interactive point control also opens up new application possibilities that go beyond the mere colorization of line art. For example, MangaNinja allows the colorization of multiple characters in a scene using different reference images or the harmonization of colors from multiple reference images. This flexibility makes MangaNinja a versatile tool for artists and designers.
Future Perspectives
MangaNinja represents an important advance in the field of AI-powered line art colorization. The precise color transfer and interactive control open up new possibilities for creative applications. Future research could focus on further improving accuracy and expanding the application possibilities, for example, by integrating semantic image analysis or supporting additional image formats.
Further Information
Further information about MangaNinja, including the source code and examples, can be found on the project page: https://johanan528.github.io/MangaNinjia/
Bibliography: - https://www.chatpaper.com/chatpaper/fr?id=4&date=1736870400&page=1 - https://www.researchgate.net/publication/358116946_Deep_Line_Art_Video_Colorization_with_a_Few_References - https://arxiv.org/pdf/2303.11137 - https://openaccess.thecvf.com/content_ICCV_2019/papers/Kim_Tag2Pix_Line_Art_Colorization_Using_Text_Tag_With_SECat_and_ICCV_2019_paper.pdf - https://diglib.eg.org/bitstream/handle/10.1111/cgf14396/v40i7pp001-010.pdf - https://ietresearch.onlinelibrary.wiley.com/doi/10.1049/ipr2.12343 - http://arxiv.org/pdf/1908.05840 - https://www.researchgate.net/figure/PaintsTorch-guided-colorization-on-line-art-PaintsTorch-takes-two-inputs-a-grayscale_fig1_337687114 - https://www.scitepress.org/Papers/2020/89614/89614.pdf - https://link.springer.com/content/pdf/10.1007/s41095-024-0414-4.pdf