Geo4D Reconstructs 4D Scenes from Videos Using Diffusion Models
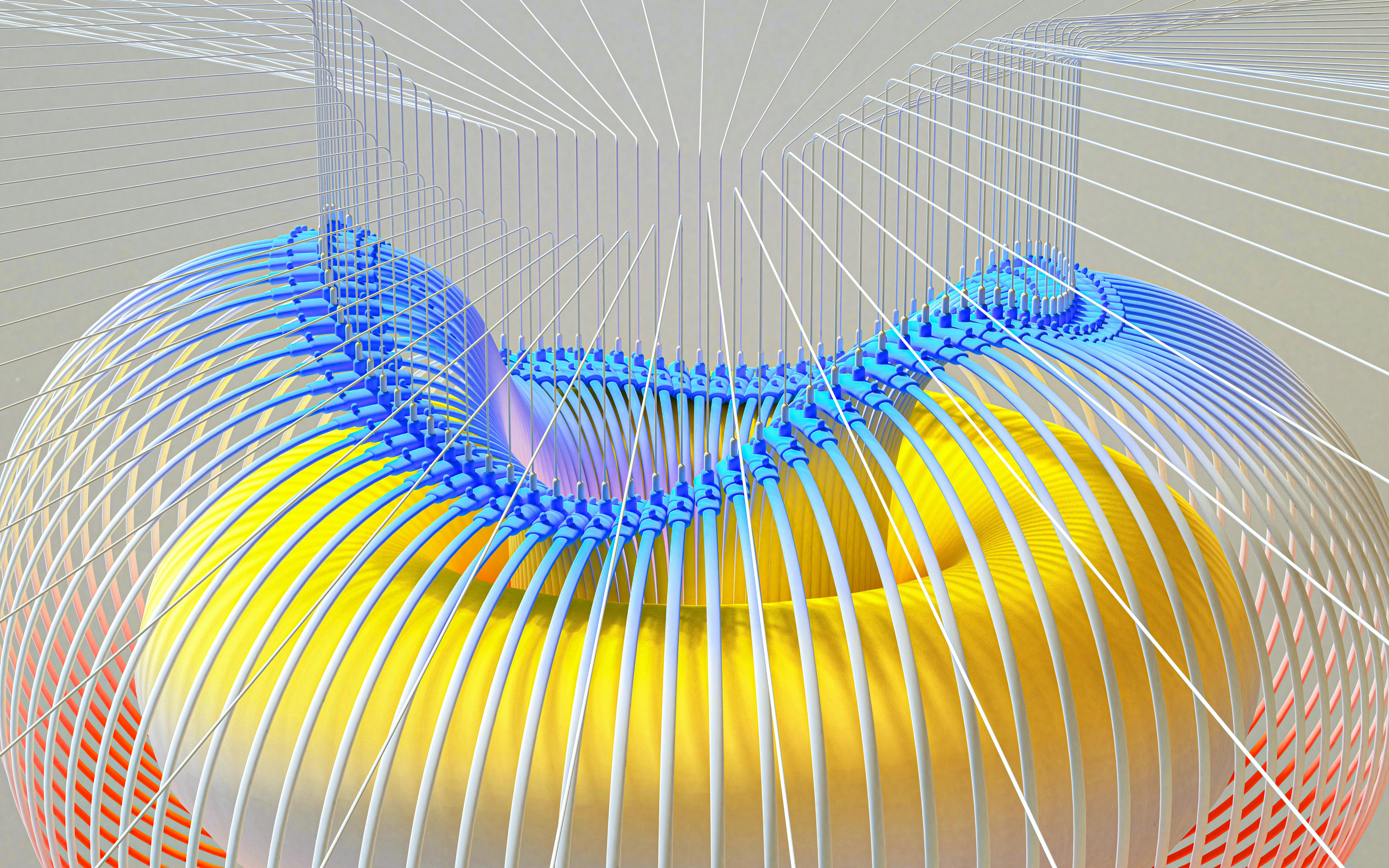
Top post
From Video Generators to 3D Worlds: Geo4D Enables Geometric 4D Scene Reconstruction
The reconstruction of three-dimensional scenes from two-dimensional images, particularly videos, is a central challenge in computer vision. A promising new approach called Geo4D leverages the power of video diffusion models to reconstruct dynamic scenes in 4D. Geo4D thus opens up new possibilities for applications in areas such as robotics, autonomous driving, augmented reality, and virtual reality.
How Geo4D Works
Geo4D is based on the principle of using pre-trained video diffusion models for 3D reconstruction. These models were originally developed to generate realistic videos. However, Geo4D utilizes their implicit understanding of three-dimensional structures and movements to extract geometric information from individual videos. In doing so, various geometric modalities are predicted, including point clouds, depth maps, and so-called ray maps, which describe the direction of light rays.
An innovative aspect of Geo4D is the use of a multimodal alignment algorithm. This algorithm allows the different predicted modalities to be aligned and fused. By combining the strengths of the individual modalities, Geo4D achieves a more robust and accurate 4D reconstruction. In addition, Geo4D uses sliding windows to effectively process even longer video sequences.
Training and Zero-Shot Generalization
Remarkably, Geo4D is trained exclusively with synthetic data. This means that no elaborate annotations of real data are required. Despite training with synthetic data, Geo4D demonstrates an impressive ability for zero-shot generalization. This means that the model is able to achieve good results even on real data that it has not seen during training.
Performance and Comparison with Other Methods
In extensive experiments on various benchmarks, Geo4D has proven its performance. The results show that Geo4D significantly outperforms the current state of the art in video depth estimation. This also applies in comparison to modern methods such as MonST3R, which were also developed for dynamic scenes. Geo4D also achieves comparable results to leading methods in camera pose estimation.
Potential Applications and Future Developments
The ability to create detailed 4D reconstructions from monocular videos opens up a wide range of application possibilities. In robotics, for example, Geo4D can be used for navigation and manipulation of objects in dynamic environments. In the field of autonomous driving, the technology can contribute to environment perception and the prediction of movements of other road users. Furthermore, Geo4D offers potential for applications in augmented and virtual reality by enabling the creation of immersive 3D experiences.
Future research could focus on improving the accuracy and robustness of Geo4D, especially in complex scenarios with occlusions and fast movements. Extending the model to other modalities, such as semantic segmentation, could also open up interesting perspectives.