Next-Generation Video Understanding with Slow-Fast Multimodal Language Models
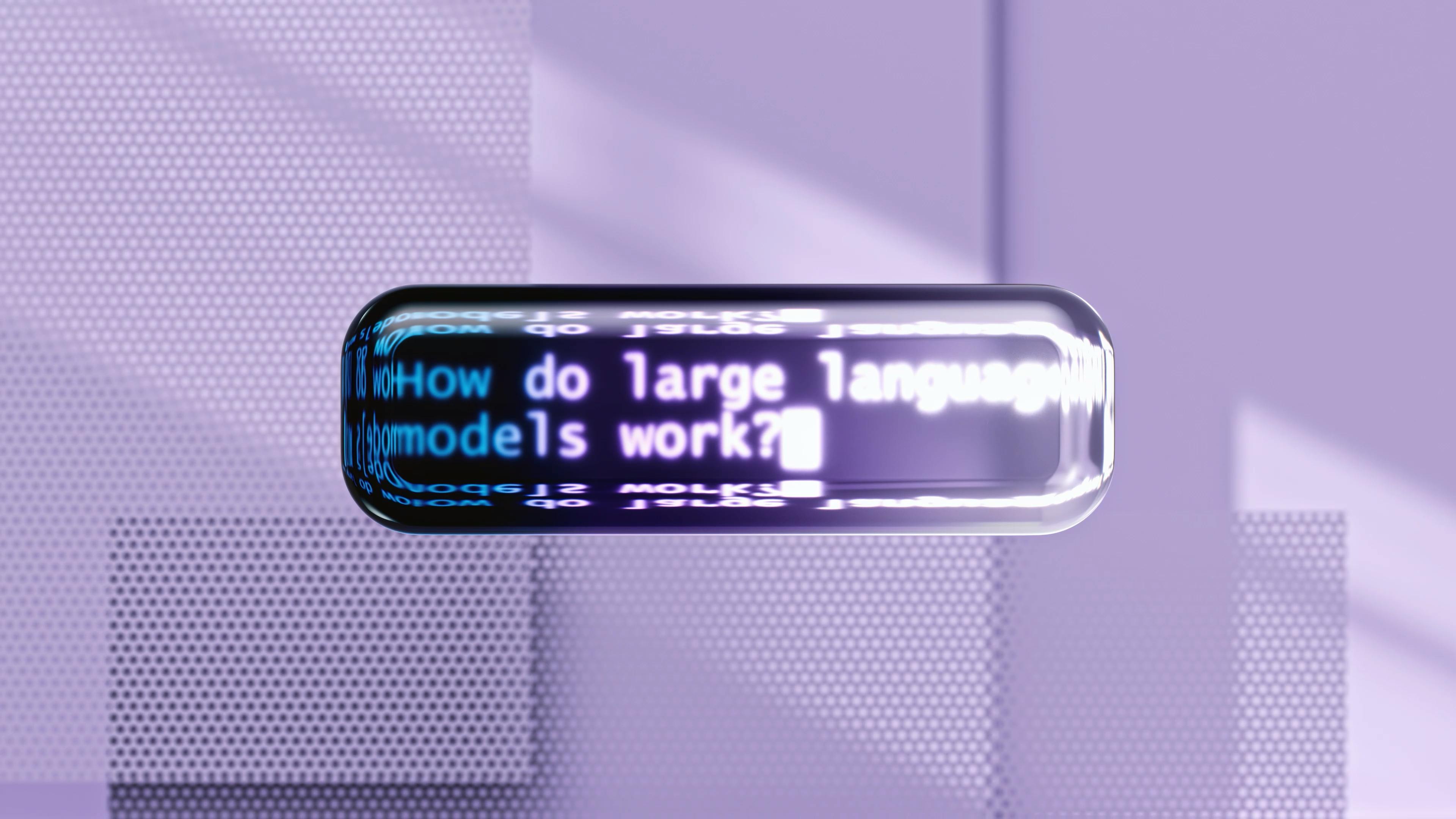
Top post
Next-Generation Video Understanding: Multimodal Language Models with Slow-Fast Architecture
The world of Artificial Intelligence (AI) is evolving rapidly, and exciting advancements are emerging in the field of video understanding. Multimodal Large Language Models (MLLMs), capable of processing both text and visual information, open up new possibilities for analyzing and interpreting videos. A particularly promising approach is the "Slow-Fast" architecture, which efficiently captures the temporal dynamics of videos.
The Challenge of Video Understanding
Videos are complex data streams containing both spatial and temporal information. Traditional methods for video analysis struggled to capture these two aspects simultaneously. While spatial information describes the "what" in an image, temporal information refers to the "how" – the movement, the change, and the sequence of events. The challenge lies in developing a model that can process both efficiently and accurately.
The Slow-Fast Architecture: Two Speeds for Optimal Performance
The Slow-Fast architecture addresses this challenge by using two separate pathways for processing visual information: a "slow" pathway and a "fast" pathway. The slow pathway processes a smaller number of frames per second and focuses on detailed spatial information. The fast pathway, on the other hand, processes a higher frame rate and captures the rapid movements and changes in the video. By combining the information from both pathways, the model gains a comprehensive understanding of the video content.
Advantages of the Slow-Fast Architecture for MLLMs
Integrating the Slow-Fast architecture into multimodal language models offers several advantages. Firstly, it allows for more efficient processing of long videos, as the temporal information is captured in a compressed manner. Secondly, it improves the accuracy of video analysis by considering both spatial details and fast movements. This opens up new possibilities for applications such as video captioning, video question answering, and the automatic generation of video summaries.
Applications and Future Perspectives
The Slow-Fast architecture for MLLMs has the potential to revolutionize various industries. In the media industry, it can enable automatic indexing and tagging of video archives. In the education sector, interactive learning platforms can emerge that intelligently analyze videos and offer personalized learning content. In the security sector, the technology can be used for the automatic detection of events and anomalies in surveillance videos. Research in this area is progressing rapidly, and it is expected that even more powerful and efficient MLLMs with Slow-Fast architecture will be developed in the future.
From Research to Practice: Mindverse as an Innovation Driver
Mindverse, a German company specializing in AI-powered content creation and analysis, recognizes the potential of this technology and is working on developing customized solutions. From chatbots and voicebots to AI search engines and knowledge bases, to individual solutions for specific use cases – Mindverse is driving innovation in the field of multimodal language models and enabling companies to leverage the benefits of this technology.
Bibliographie: Feichtenhofer, C., Pinheiro, P., & Zisserman, A. (n.d.). A Slow-I-Fast-P Architecture for Compressed Video Action Recognition. Fu, B. (n.d.). Awesome-Multimodal-Large-Language-Models. Shao, H., Chen, S., Wang, B., Chang, S.-F., & Li, F.-F. (2024). SlowFast-LLaVA-15: A Family of Token-Efficient Video Large Language Models for Long-Form Video Understanding. Wu, C., Wu, F., Chen, T., Wang, X., Zhu, Y., & Xie, X. (2025). Visual Instruction Tuning. Yao, T., Huang, D., & Sun, C. (n.d.). FP-DETR: Detection Transformer Advanced by Feature Pyramid. Zhou, Y., Wang, J., & Vaswani, A. (n.d.). Co-Training Multi-Modal Large Language Models.