AI and Robotics Accelerate Scientific Discovery Through Scaling Laws
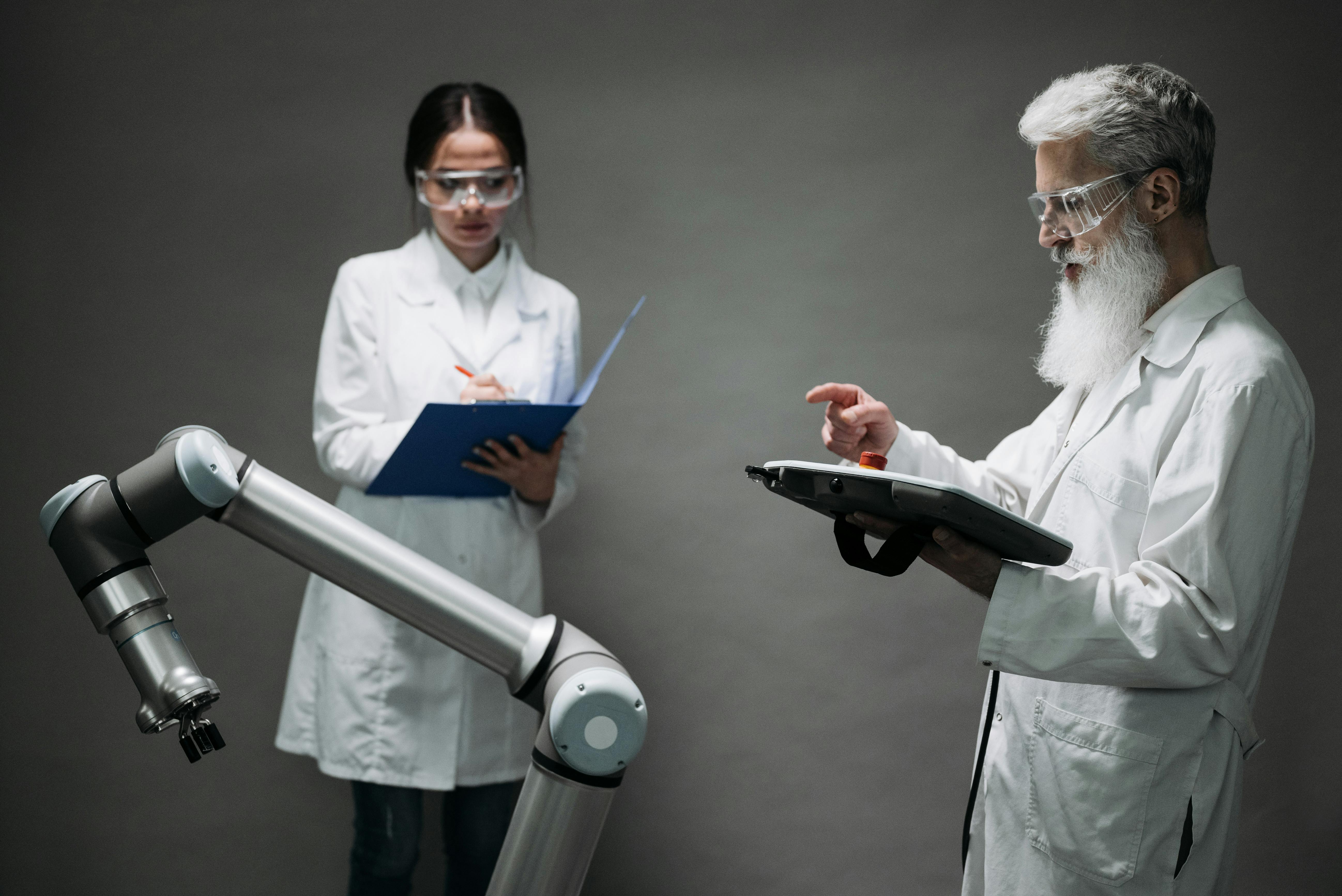
Top post
Scaling Laws: How AI and Robots Accelerate Scientific Research
Scientific discovery is a complex and often lengthy process. Traditionally shaped by human intuition and laborious laboratory work, research is currently undergoing a profound transformation through the advent of Artificial Intelligence (AI) and robotics. A particularly promising area is the exploration of so-called scaling laws, which have the potential to significantly increase the speed and efficiency of scientific breakthroughs.
Scaling laws describe how the performance of an AI system changes with increasing computing power, data volume, and model size. In scientific research, these laws can be used to predict and optimize progress in various disciplines. For example, AI models can be trained to analyze huge datasets from experiments, recognize patterns, and generate hypotheses that can then be tested by robots in the laboratory.
Robot scientists, equipped with AI-driven algorithms, can perform experiments automatically, collect data precisely, and document results reproducibly. This frees human researchers from repetitive tasks and allows them to focus on interpreting the results and developing new research questions. The combination of AI and robotics makes it possible to conduct experiments on a much larger scale and at a higher speed than would be possible with traditional methods.
From Data Analysis to Hypothesis Generation
AI-powered systems can analyze complex scientific data, such as genome sequences or climate models, and uncover hidden relationships within them. Through machine learning, these systems can recognize patterns that remain hidden to the human eye, thus providing new insights into biological processes or environmental changes. Furthermore, AI models can be used to generate hypotheses and suggest new research approaches that can be pursued by human scientists.
Automated Experiments and Reproducible Results
Robot scientists can automatically perform experiments designed by AI systems. They can take precise measurements, collect data, and document the results. The automation of experiments reduces the risk of human error and increases the reproducibility of results. This is particularly important in areas such as drug development or materials research, where precise and reliable data are essential.
Challenges and Opportunities
The application of scaling laws in scientific research also presents challenges. The development of robust and reliable AI models requires large amounts of data and high computing power. In addition, the ethical and societal implications of automating research processes must be carefully considered. Despite these challenges, the combination of AI and robotics offers enormous opportunities for scientific discovery. By accelerating research processes and enabling new insights, we can find solutions to pressing global problems such as climate change, diseases, and resource scarcity.
Research on scaling laws in scientific discovery is still in its early stages, but the results so far are promising. With increasing computing power and improved AI algorithms, the importance of AI and robotics in research will continue to grow. The future of scientific discovery will be shaped by intelligent collaboration between humans and machines.
Bibliographie: - https://arxiv.org/abs/2503.22444 - https://arxiv.org/html/2503.22444v1 - https://chatpaper.com/chatpaper/fr/paper/124993 - https://paperreading.club/page?id=295886 - https://www.zhuanzhi.ai/paper/37adbe50f8efdbcdc7ad0550bf38c627 - https://www.researchgate.net/figure/Scaling-laws-of-scientific-discovery-from-humans-through-co-scientists-to-AGS-breaking_fig3_383213197 - https://www.cs.cornell.edu/gomes/pdf/2023_wang_nature_aisci.pdf - https://www.linkedin.com/posts/sebastian-sartor_neural-scaling-laws-for-embodied-ai-activity-7199734381026979842-LUSS - https://openreview.net/pdf?id=SskZeP3Mzz - https://www.pnas.org/doi/10.1073/pnas.2401238121